Medical scanner teaching itself to optimize clinical protocols and image acquisition
A technology of medical images and scanners, applied in the field of medical scanners
- Summary
- Abstract
- Description
- Claims
- Application Information
AI Technical Summary
Problems solved by technology
Method used
Image
Examples
Embodiment Construction
[0016] Systems, methods, and apparatus are described herein that relate generally to various techniques related to self-learning systems for medical scanners that automatically learn to optimize image acquisition. More specifically, the techniques described herein optimize image acquisition at a medical scanner using a self-learning model of operator parameter selection utilizing parameters available, for example, locally at the scanner or in a connected database. Collected parameters may include, for example, image data, patient data, attributes from clinical protocols and scanner parameters, and configurations. The examples provided in this paper describe techniques in the context of deep reinforcement learning frameworks, where an intelligent agent identifies relevant features from a pool of attributes, and then derives actions to automatically aggregate into target parameter settings. However, the general approach described in this paper can be extended to other forms of m...
PUM
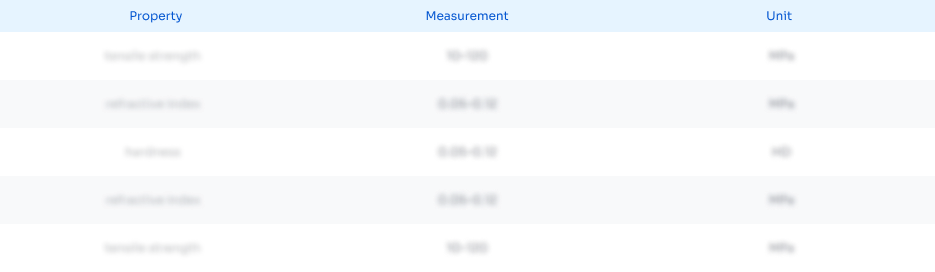
Abstract
Description
Claims
Application Information
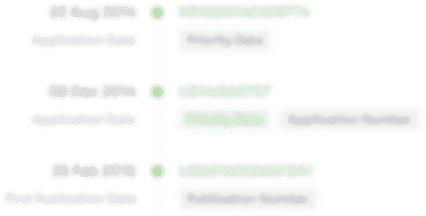
- R&D Engineer
- R&D Manager
- IP Professional
- Industry Leading Data Capabilities
- Powerful AI technology
- Patent DNA Extraction
Browse by: Latest US Patents, China's latest patents, Technical Efficacy Thesaurus, Application Domain, Technology Topic.
© 2024 PatSnap. All rights reserved.Legal|Privacy policy|Modern Slavery Act Transparency Statement|Sitemap