Improved method for learning discriminative segments in fine-grained identification
A discriminative, fine-grained technology, applied in character and pattern recognition, instruments, biological neural network models, etc., can solve problems such as expensive and difficult for ordinary people to complete, achieve reduced interference, simple and feasible practicability, and improved The effect of classification accuracy
- Summary
- Abstract
- Description
- Claims
- Application Information
AI Technical Summary
Problems solved by technology
Method used
Image
Examples
specific example
[0060] figure 1 A structural flow chart of the present invention is described. The structure of the present invention mainly consists of three parts, as figure 1 Shown in ①, ②, ③. This method is based on the VGG-16 model, which has 16 layers. The implementation process is divided into two phases: training phase and testing phase.
[0061] In the training phase, the parameters of the detector are mainly learned, and the process is as follows: figure 1 Shown in ① and ②.
[0062] (1) First, the input image passes through the pre-trained convolutional neural network VGG-16, and conv4-3 outputs a feature map with a size of 512×28×28. Therefore, the size of each detector is 512×1×1. Set the number of detectors of each type to 10, then there are 2000 detectors for the CUB200-2011 data set;
[0063] (2) Convolute each detector with the obtained 512×28×28 feature map to obtain a 28×28 size response map;
[0064] (3) A 2000-dimensional feature vector is obtained after pooling the...
PUM
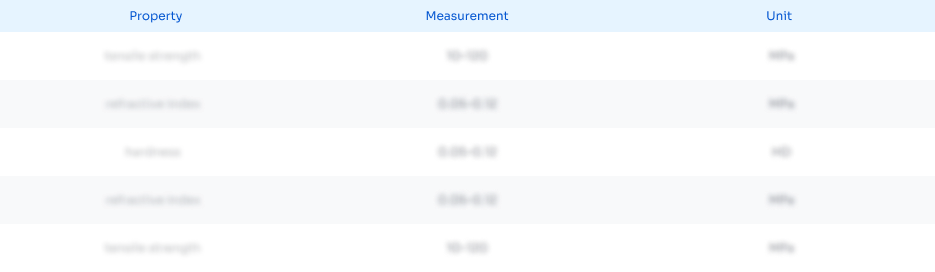
Abstract
Description
Claims
Application Information
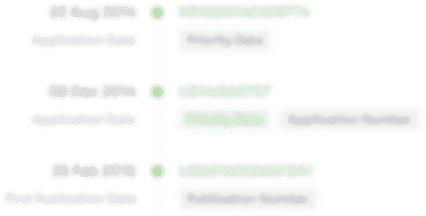
- Generate Ideas
- Intellectual Property
- Life Sciences
- Materials
- Tech Scout
- Unparalleled Data Quality
- Higher Quality Content
- 60% Fewer Hallucinations
Browse by: Latest US Patents, China's latest patents, Technical Efficacy Thesaurus, Application Domain, Technology Topic, Popular Technical Reports.
© 2025 PatSnap. All rights reserved.Legal|Privacy policy|Modern Slavery Act Transparency Statement|Sitemap|About US| Contact US: help@patsnap.com