Regression model hyperparameter optimization method
A regression model and optimization method technology, applied in the fields of electrical digital data processing, special data processing applications, instruments, etc., can solve the problems of error estimation uncertainty, increasing computational complexity, powerlessness, etc.
- Summary
- Abstract
- Description
- Claims
- Application Information
AI Technical Summary
Problems solved by technology
Method used
Image
Examples
Embodiment Construction
[0027] The present invention will be further described in detail with reference to the accompanying drawings and embodiments.
[0028] Hyperparameter optimization problem: Known regression dataset {(x i ,y i ), i=1,2,...,n} and the regression model m(·|p), for the hyperparameter p of the model, L candidate parameters {p l , l=1,2,...,L} select a parameter p* that is most suitable for this data set, that is, to make the regression model m(·|p*) achieve the best prediction accuracy on this data set .
[0029] Symbolic representation: {(x i ,y i ), i=1,2,…,n} represent the existing regression data set, where x i ,y i Represent the input vector and output value of the i-th sample, n is the sample size in the data set; m(·|p) represents the regression model, where p is the hyperparameter of the model, and its value range is a set containing L elements The set of arithmetic difference or geometric sequence P={p l ,l=1,2,...,L}; p* is the optimal hyperparameter, and p*∈P; the...
PUM
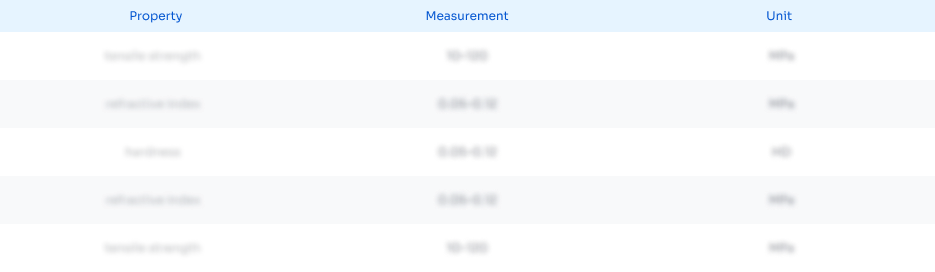
Abstract
Description
Claims
Application Information
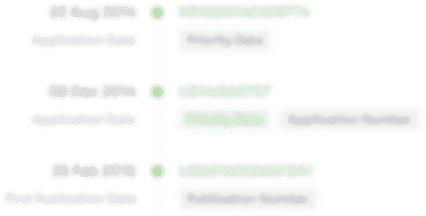
- R&D Engineer
- R&D Manager
- IP Professional
- Industry Leading Data Capabilities
- Powerful AI technology
- Patent DNA Extraction
Browse by: Latest US Patents, China's latest patents, Technical Efficacy Thesaurus, Application Domain, Technology Topic, Popular Technical Reports.
© 2024 PatSnap. All rights reserved.Legal|Privacy policy|Modern Slavery Act Transparency Statement|Sitemap|About US| Contact US: help@patsnap.com