Recurrent neural network method for improving time sequence data mining capability of recommendation model
A cyclic neural network and time series data technology, applied in neural learning methods, biological neural network models, neural architectures, etc., can solve the problem of failing to directly use the time information of sequence data, and achieve the effect of improving mining ability and accuracy.
- Summary
- Abstract
- Description
- Claims
- Application Information
AI Technical Summary
Problems solved by technology
Method used
Image
Examples
Embodiment Construction
[0051] In order to make the purpose, technical solution and advantages of the present invention clearer, a model combining cyclic neural network structures such as LSTM and time gates will be used below (for the convenience of description, Time-LSTM will be used to refer to the combination model hereinafter) to The time gate is further described in terms of specific functions.
[0052] Such as figure 1 As shown, it is a flow chart of the cyclic neural network method for improving the time-series data mining ability of the recommendation model in the present invention.
[0053] First, according to step 1, modify the traditional neural network and add time gate components to the network.
[0054] In order to modify a traditional neural network, a brief overview of its basic model is first given. Such as figure 2 As shown, the basic LSTM model has three gate structures: input gate, forget gate, and output gate.
[0055] Input gate: The role of the input gate is to determine ...
PUM
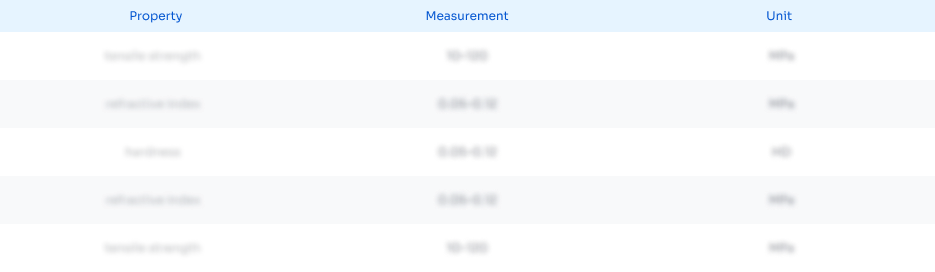
Abstract
Description
Claims
Application Information
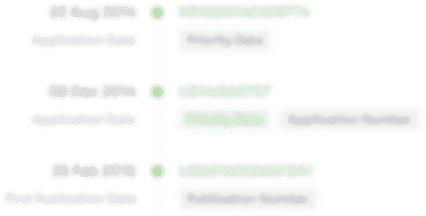
- R&D Engineer
- R&D Manager
- IP Professional
- Industry Leading Data Capabilities
- Powerful AI technology
- Patent DNA Extraction
Browse by: Latest US Patents, China's latest patents, Technical Efficacy Thesaurus, Application Domain, Technology Topic, Popular Technical Reports.
© 2024 PatSnap. All rights reserved.Legal|Privacy policy|Modern Slavery Act Transparency Statement|Sitemap|About US| Contact US: help@patsnap.com