Face super-resolution reconstruction method based on deep learning
A technology of super-resolution reconstruction and deep learning, applied in image data processing, instrumentation, computing, etc., can solve problems such as low resolution, out-of-focus blur, and motion blur
- Summary
- Abstract
- Description
- Claims
- Application Information
AI Technical Summary
Problems solved by technology
Method used
Image
Examples
Embodiment Construction
[0040] Such as figure 1 As shown, the present invention discloses a face super-resolution reconstruction method based on deep learning. The specific implementation of the present invention will be described in detail below in conjunction with the accompanying drawings.
[0041] Step 1: Use the Multi-task convolutional neural networks (MTCNN) algorithm to extract 5 key points of the face. The face data uses the CelebA dataset, which contains 202,599 pictures of 10,177 individuals. The MTCNN algorithm is composed of three network structures (P-Net, R-Net, O-Net), wherein P-Net (Propsoal Network) mainly obtains candidate windows and frame regression vectors of the face area; R-Net (Refine Network) obtains more accurate candidate windows on the basis of P-Net; O-Net (Output Network) further improves the window accuracy on the basis of R-Net, and outputs 5 key point coordinates at the same time. The coordinates of the five key points include the coordinates of the left eye, the ri...
PUM
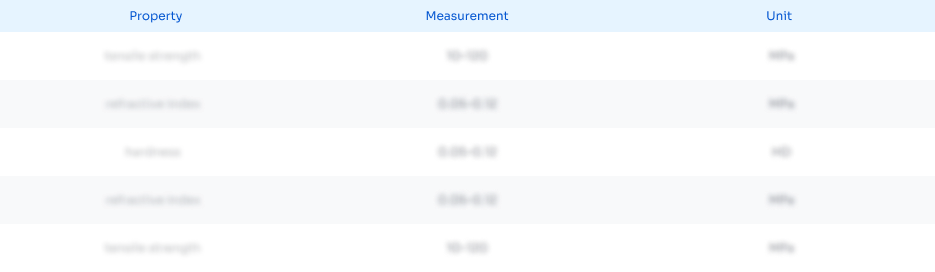
Abstract
Description
Claims
Application Information
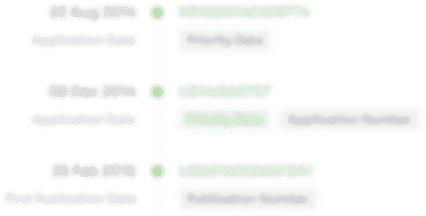
- R&D Engineer
- R&D Manager
- IP Professional
- Industry Leading Data Capabilities
- Powerful AI technology
- Patent DNA Extraction
Browse by: Latest US Patents, China's latest patents, Technical Efficacy Thesaurus, Application Domain, Technology Topic, Popular Technical Reports.
© 2024 PatSnap. All rights reserved.Legal|Privacy policy|Modern Slavery Act Transparency Statement|Sitemap|About US| Contact US: help@patsnap.com