An Image Recognition Method Based on Variational Group Convolution
An image recognition and convolution technology, applied in the field of image recognition of variable group convolution, can solve the problem of overfitting without considering the input channel, reduce the possibility of overfitting, be easy to implement, and improve the degree of generalization Effect
- Summary
- Abstract
- Description
- Claims
- Application Information
AI Technical Summary
Problems solved by technology
Method used
Image
Examples
Embodiment Construction
[0024] The present invention is described in detail below in conjunction with accompanying drawing and embodiment,
[0025] Such as figure 1 Shown, the concrete realization steps of the present invention are as follows:
[0026] (1) For each convolution kernel in m convolution kernels (the number of m can be adjusted manually, it is recommended that m The combination of , assuming a total of n' combined feature maps, that is,
[0027]
[0028] The feature maps of m combinations are randomly selected from these n' combinations, and each such combination corresponds to a convolution kernel, and there are m convolution kernels in total.
[0029] (2) For the m combined feature maps and corresponding m convolution kernels randomly extracted in the second step, it is assumed that the i-th convolution kernel among the m convolution kernels of the current l layer corresponds to k input features , the k feature maps are convolved,
[0030]
[0031] where W i Is the convolut...
PUM
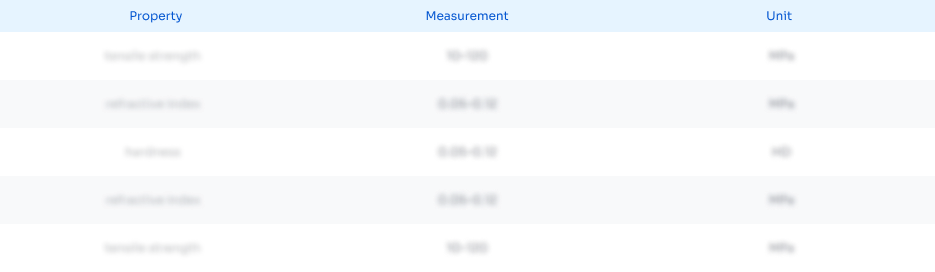
Abstract
Description
Claims
Application Information
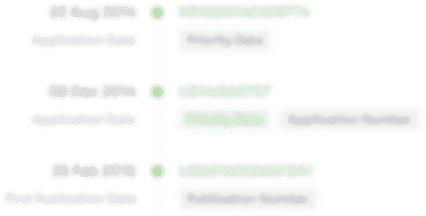
- R&D Engineer
- R&D Manager
- IP Professional
- Industry Leading Data Capabilities
- Powerful AI technology
- Patent DNA Extraction
Browse by: Latest US Patents, China's latest patents, Technical Efficacy Thesaurus, Application Domain, Technology Topic, Popular Technical Reports.
© 2024 PatSnap. All rights reserved.Legal|Privacy policy|Modern Slavery Act Transparency Statement|Sitemap|About US| Contact US: help@patsnap.com