A Two-Stage Recognition Method Based on Non-Negative Representation Coefficients
A non-negative technology for expressing coefficients, applied in the field of machine learning, can solve problems such as slow calculation speed, long time-consuming, complicated calculation process, etc., and achieve the effects of fast running speed, accurate classification results, and accurate recognition
- Summary
- Abstract
- Description
- Claims
- Application Information
AI Technical Summary
Problems solved by technology
Method used
Image
Examples
Embodiment
[0017] In this embodiment, the FERET face database is used as the experimental data. The FERET face database is a database containing 200 people and 7 face images per person. In order to verify the effectiveness and practicability of the present invention, the present invention respectively selects the first m=1, 2, 3, 4, 5 images of each person as training samples, and uses the remaining 7-m images of each person as test samples , so the total number of training samples is 200×m, and the total number of test samples is 200×(7-m). The first seven images of a face as a training sample in this embodiment are as follows: figure 1 shown.
[0018] In this embodiment, the following definitions are made:
[0019] let x ij is a p-dimensional column vector and represents the j-th original training sample of the i-th class, i=1,2,...,c,j=1,2,...,n i , where n i is the number of training samples for each class, N=n 1 +n 2 +…+n c is the total number of training samples, training ...
PUM
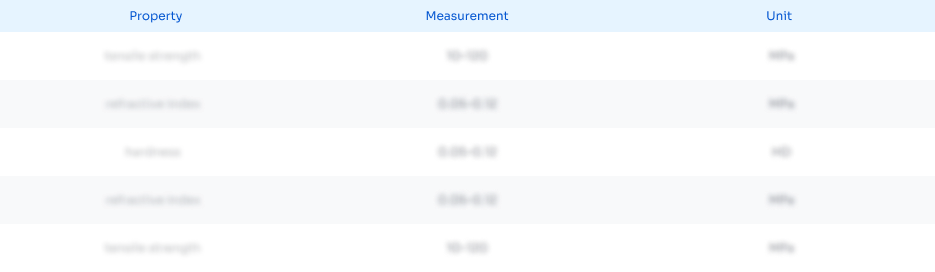
Abstract
Description
Claims
Application Information
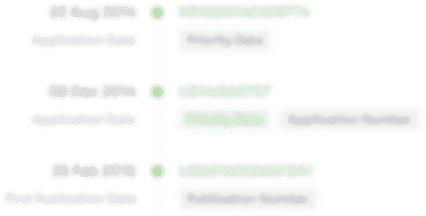
- R&D Engineer
- R&D Manager
- IP Professional
- Industry Leading Data Capabilities
- Powerful AI technology
- Patent DNA Extraction
Browse by: Latest US Patents, China's latest patents, Technical Efficacy Thesaurus, Application Domain, Technology Topic.
© 2024 PatSnap. All rights reserved.Legal|Privacy policy|Modern Slavery Act Transparency Statement|Sitemap