Multi-scale feature fusion ultrasonic image semantic segmentation method based on adversarial learning
A multi-scale feature and semantic segmentation technology, applied in the field of medical image understanding, can solve the problems of reduced resolution of feature maps, failure to consider similarity of pixel features, and insufficient use of local features and global context features.
- Summary
- Abstract
- Description
- Claims
- Application Information
AI Technical Summary
Problems solved by technology
Method used
Image
Examples
Embodiment approach
[0077] It can be derived from Table 2 that the size of the convolution kernel of each anti-convolutional layer is 5×5, and the step size is 2, and the number of convolution kernels of the first to sixth anti-convolutional layers is 32 in order. , 64, 128, 256, 512, 1024; The sizes of the first to third fully connected layers are 1024, 512, and 2, respectively, where 2 represents the two categories of whether the input image comes from a segmentation network or a segmentation label. Specifically, the input of the adversarial identification network is 2 channels, which respectively represent the probability distribution maps of pixels belonging to two categories of normal and lesions. Among them, the segmentation map and segmentation label of each pair of images (B-mode image and elastic image) correspond to two A probability distribution map, one is the probability distribution map of each pixel belonging to the normal tissue, and the other is the probability distribution map of ...
PUM
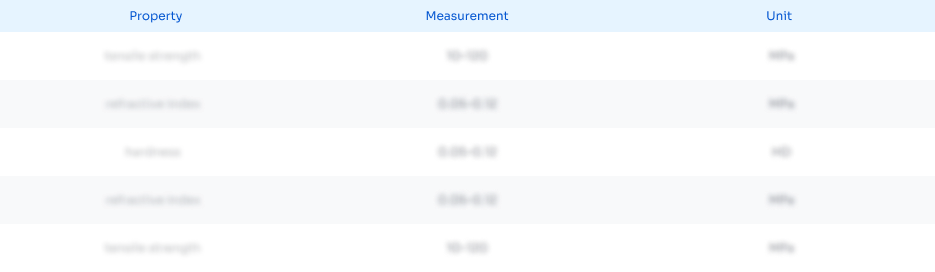
Abstract
Description
Claims
Application Information
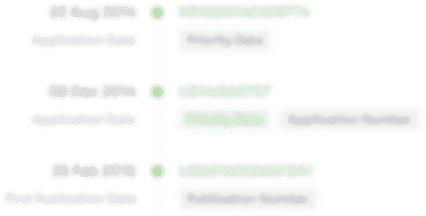
- R&D
- Intellectual Property
- Life Sciences
- Materials
- Tech Scout
- Unparalleled Data Quality
- Higher Quality Content
- 60% Fewer Hallucinations
Browse by: Latest US Patents, China's latest patents, Technical Efficacy Thesaurus, Application Domain, Technology Topic, Popular Technical Reports.
© 2025 PatSnap. All rights reserved.Legal|Privacy policy|Modern Slavery Act Transparency Statement|Sitemap|About US| Contact US: help@patsnap.com