Paper defect classification method based on multi-scale morphology combined with convolutional neural network
A convolutional neural network and defect classification technology, applied in the field of paper defect classification combined with multi-scale morphology and convolutional neural network, can solve the problems of simple texture, single background, single underlying feature, etc., achieving high accuracy and low time consumption Effect
- Summary
- Abstract
- Description
- Claims
- Application Information
AI Technical Summary
Problems solved by technology
Method used
Image
Examples
Embodiment 1
[0053] In order to test the effectiveness and superiority of the present invention for classifying paper defect images, the simulation experiments are carried out under the hardware environment of CPU: Intel(TM) i7-6700U, 3.3GHz, memory 16GB, NVIDIA Quadro K620 graphics card and software environment of MATLABR2017a of.
[0054] Three comparison methods are used: the traditional method extracts the HOG feature of paper defects for classification, and obtains the classification result (HOG+SVM), and extracts the LBP feature for classification to obtain the classification result (LBP+SVM). The classification results obtained by inputting the image to the CNN model after image enhancement (Canny+CNN), the classification results obtained by the Sobel operator for four types of paper defects after image enhancement and input to the CNN model (Sobel+CNN), and the Prewitt operator for the four types of paper defects The classification result (Prewitt+CNN) obtained by inputting the ima...
PUM
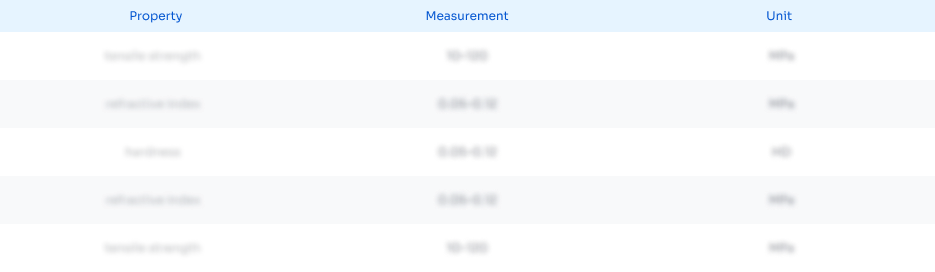
Abstract
Description
Claims
Application Information
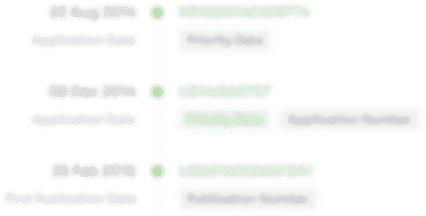
- R&D
- Intellectual Property
- Life Sciences
- Materials
- Tech Scout
- Unparalleled Data Quality
- Higher Quality Content
- 60% Fewer Hallucinations
Browse by: Latest US Patents, China's latest patents, Technical Efficacy Thesaurus, Application Domain, Technology Topic, Popular Technical Reports.
© 2025 PatSnap. All rights reserved.Legal|Privacy policy|Modern Slavery Act Transparency Statement|Sitemap|About US| Contact US: help@patsnap.com