Air quality prediction method based on deep neural network
A deep neural network, air quality technology, applied in the field of air quality prediction, can solve the problems of lack of physical foundation, low accuracy of prediction results, difficult to give boundary and initial conditions, etc., to achieve the effect of improving the quality of prediction
- Summary
- Abstract
- Description
- Claims
- Application Information
AI Technical Summary
Problems solved by technology
Method used
Image
Examples
Embodiment
[0028] Such as figure 1 Shown, the present invention relates to a kind of air quality prediction method based on deep neural network, and this method comprises the following steps:
[0029] Step 1. Determine the time series and time points at equal intervals, and collect the concentration of each air pollutant at the time points at equal intervals at different locations;
[0030] Step 2. Sorting the concentration of each pollutant collected, obtaining concentration vectors at different time points at different locations, and the concentration types in each concentration vector are sorted in the same order;
[0031] Step 3, use the concentration vectors at different locations at the same time point as a data group, and use all data groups in a time series as a set;
[0032] Step 4, selecting a known set containing the concentration values of all pollutants from multiple sets, and judging the time point corresponding to the missing concentration data group in the set to be te...
PUM
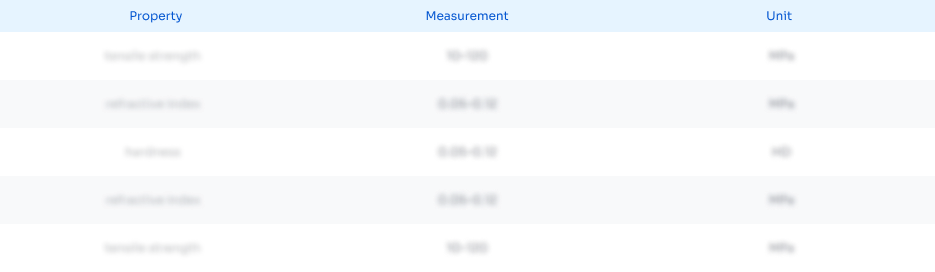
Abstract
Description
Claims
Application Information
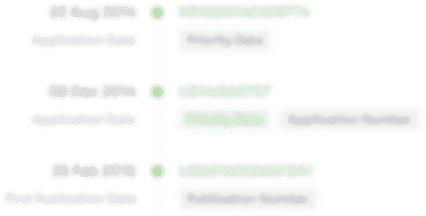
- R&D Engineer
- R&D Manager
- IP Professional
- Industry Leading Data Capabilities
- Powerful AI technology
- Patent DNA Extraction
Browse by: Latest US Patents, China's latest patents, Technical Efficacy Thesaurus, Application Domain, Technology Topic.
© 2024 PatSnap. All rights reserved.Legal|Privacy policy|Modern Slavery Act Transparency Statement|Sitemap