Power load prediction method based on sparse representation
A technology of power load and forecasting method, which is applied in the field of power load forecasting based on sparse representation, can solve the problem of poor ability to learn and process uncertainty and artificial information, cannot truly reflect the nonlinear characteristics of the load model, and cannot be very good. Adaptation and other problems to achieve the effect of concise and clear data structure, insensitive to noise, and not easy to over-fit
- Summary
- Abstract
- Description
- Claims
- Application Information
AI Technical Summary
Problems solved by technology
Method used
Image
Examples
Embodiment Construction
[0027] The present invention will be further described below in conjunction with the accompanying drawings and embodiments.
[0028] Please refer to figure 1 , the present invention provides a kind of electric load forecasting method based on sparse representation, it is characterized in that: comprise the following steps:
[0029] Step S1: Obtain the real power load data y through the power company and perform preprocessing operations on it to obtain preprocessed power load data y′. The preprocessing operations include data missing, data abnormality and data normalization;
[0030] Step S2: Build an overcomplete dictionary: Construct the basic dictionary, the identity matrix dictionary generated by the delta function, and the external factor dictionary composed of external factors by splicing discrete wavelet transform or discrete residual transform to form an overcomplete dictionary D, The overcomplete dictionary is divided into two parts D1 is the training dictionary, wh...
PUM
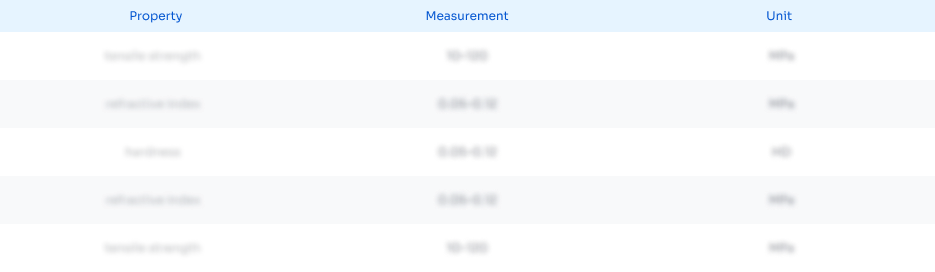
Abstract
Description
Claims
Application Information
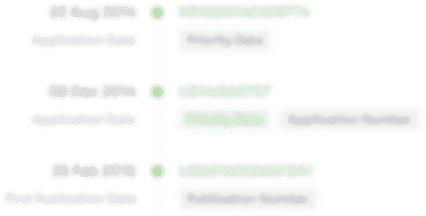
- R&D Engineer
- R&D Manager
- IP Professional
- Industry Leading Data Capabilities
- Powerful AI technology
- Patent DNA Extraction
Browse by: Latest US Patents, China's latest patents, Technical Efficacy Thesaurus, Application Domain, Technology Topic.
© 2024 PatSnap. All rights reserved.Legal|Privacy policy|Modern Slavery Act Transparency Statement|Sitemap