Full convolutional neural network-based large-range remote sensing image semantic segmentation method
A convolutional neural network and remote sensing image technology, applied in the field of semantic segmentation of large-scale remote sensing images, can solve problems affecting use, achieve high precision, and reduce manual workload
- Summary
- Abstract
- Description
- Claims
- Application Information
AI Technical Summary
Problems solved by technology
Method used
Image
Examples
Embodiment Construction
[0039] In order to describe the present invention more specifically, the technical solutions of the present invention will be described in detail below in conjunction with the accompanying drawings and specific embodiments.
[0040] Such as figure 1 As shown, the present invention is based on a full convolutional neural network semantic segmentation method for large-scale remote sensing images, including the following steps:
[0041] (1) Remote sensing image fusion and band synthesis.
[0042] In the training of machine learning, the quality and quantity of samples are very important; in practical application scenarios, the number of samples can usually be satisfied by some techniques, but the quality of samples depends entirely on manual labeling. Because it is manually labeled, a certain amount of data that has not been correctly labeled is often included in the training. Such data will inevitably have some negative effects on the final result; in order to minimize the nega...
PUM
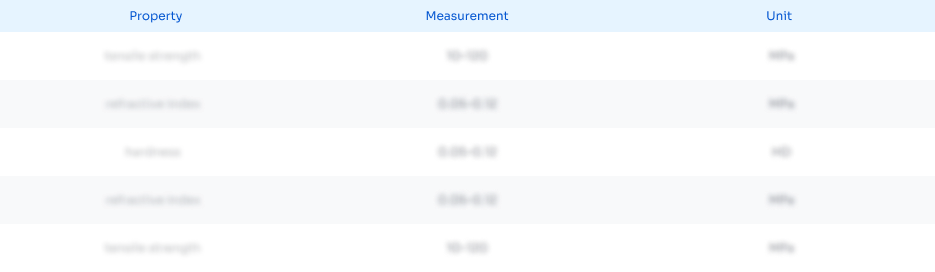
Abstract
Description
Claims
Application Information
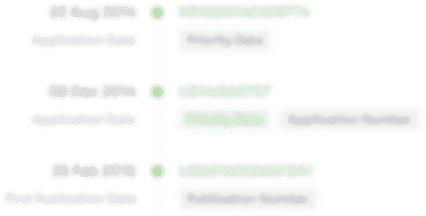
- R&D Engineer
- R&D Manager
- IP Professional
- Industry Leading Data Capabilities
- Powerful AI technology
- Patent DNA Extraction
Browse by: Latest US Patents, China's latest patents, Technical Efficacy Thesaurus, Application Domain, Technology Topic, Popular Technical Reports.
© 2024 PatSnap. All rights reserved.Legal|Privacy policy|Modern Slavery Act Transparency Statement|Sitemap|About US| Contact US: help@patsnap.com