Deep learning based time sequence motion identification method
An action recognition and deep learning technology, applied in the fields of computer vision and pattern recognition, can solve the problems of inaccurate regression of long action boundaries and insufficient validity of long action feature expression, and achieve the recognition rate of sequential actions, clear action time boundaries, The effect of reducing the amount of subsequent calculations
- Summary
- Abstract
- Description
- Claims
- Application Information
AI Technical Summary
Problems solved by technology
Method used
Image
Examples
Embodiment Construction
[0039] The present invention will be described in detail below in conjunction with the accompanying drawings and specific embodiments.
[0040] In order to improve the subjective quality of the video, the present invention considers the length limit of long motions during multi-scale construction, and proposes a brand-new splicing mechanism for incomplete motion segments, which effectively improves the accuracy of long motion boundaries, and by considering contextual information, Further accurately identify action segments. The invention discloses a time series action detection method based on deep learning, the flow is as follows figure 1 as shown,
[0041] Specifically follow the steps below:
[0042] The present invention selects the temporal action detection data set THUMOS Challenge 2014 as the experimental database, which contains 20 types of undivided videos containing temporal action tags, and the present invention selects 200 of the verifier videos (including 3007 b...
PUM
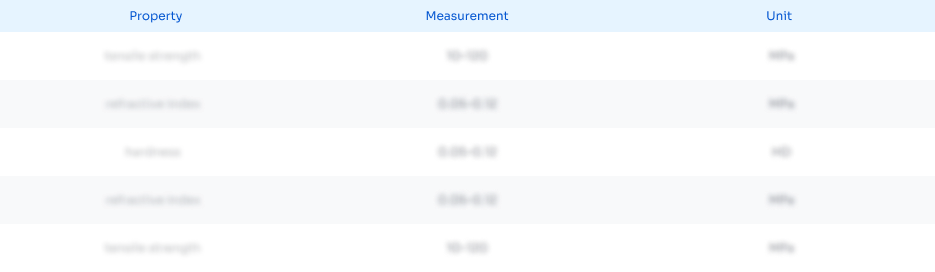
Abstract
Description
Claims
Application Information
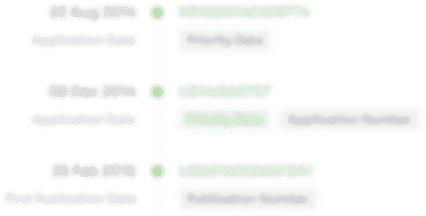
- Generate Ideas
- Intellectual Property
- Life Sciences
- Materials
- Tech Scout
- Unparalleled Data Quality
- Higher Quality Content
- 60% Fewer Hallucinations
Browse by: Latest US Patents, China's latest patents, Technical Efficacy Thesaurus, Application Domain, Technology Topic, Popular Technical Reports.
© 2025 PatSnap. All rights reserved.Legal|Privacy policy|Modern Slavery Act Transparency Statement|Sitemap|About US| Contact US: help@patsnap.com