Image style migration model training method and image style migration method
A model training and image technology, applied in the field of image processing, can solve the problems such as the inability to transfer the style of the filter, the limited types of filters, etc., and achieve the effect of controllability and speed.
- Summary
- Abstract
- Description
- Claims
- Application Information
AI Technical Summary
Problems solved by technology
Method used
Image
Examples
example 1
[0066] Example 1: Subtract the pixel values of the pixels corresponding to the R channel positions of the second feature map and the third feature map; the first feature Figure 5 The values of pixels A, B, C, D, and E in the R channel are: 235, 233, 232, 230, and 240, respectively. The values of the pixels A', B', C', D', and E' on the third feature map corresponding to the pixel positions on the second feature map in the R channel are: 125, 127, 124, 130, 132 . Subtract the pixel values of the pixels corresponding to the R channel positions of the second feature map and the third feature map, and the obtained channel difference values corresponding to each pixel point in the R channel are: 110, 106, 108, 100, and 108 .
[0067] Perform noise elimination processing on the channel difference, the process is: detect whether the channel difference corresponding to each pixel point in each channel is greater than 1, if greater than 1, calculate the channel loss of the...
example 2
[0081] Example 2: The second feature map includes three pixels A, D, and C, and the pixel values of pixel A on the three channels of R, G, and B are respectively: 255,167,220; pixel D is on the three channels of R, G, and B The pixel values on the channels are 250, 162, 221 respectively; the pixel values of pixel C on the R, G, and B channels are 240, 150, 190 respectively;
[0082] Then the average value of the pixel point A in the three color channels of R, G, and B is: (255+167+220) / 3=214;
[0083] The pixel mean value of the pixel point B in the three color channels of R, G, and B is: (250+162+221) / 3=211;
[0084] The pixel average value of the pixel point C in the three color channels of R, G, and B is: (240+150+190) / 3=193.
[0085] Assume that the pixels in the third feature map corresponding to the three pixel positions of the calculated pixels A, B, and C are: A', B', and C', and the pixel A' is in the three colors of R, G, and B The pixel mean value of the cha...
example 3
[0106] Example 3: Assume that the first feature map includes three pixels A, B, and C, and the pixel values of pixel A on the three channels of R, G, and B are respectively: 255,167,220; pixel B is on R, G, and B The pixel values on the three channels are 250, 162, and 221 respectively; the pixel values of pixel C on the R, G, and B channels are 240, 150, and 190 respectively;
[0107] To normalize the pixel values of each pixel in the first feature map in different color channels is to divide the pixel values of each pixel in the first feature map in different color channels by 255.
[0108] For example, in this example three, the normalized results of the pixel values of pixel A on the three channels of R, G, and B are respectively: 255 / 255, 167 / 255, 220 / 255; The normalized results of the pixel values on the channels are: 250 / 255, 162 / 255, 221 / 255; the normalized results of the pixel values of pixel C on the R, G, and B channels are: 240 / 255, 150 / 255, 190 / 2...
PUM
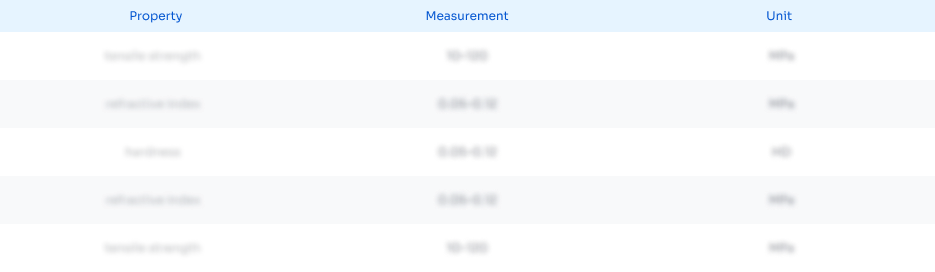
Abstract
Description
Claims
Application Information
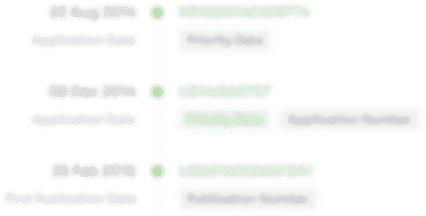
- R&D Engineer
- R&D Manager
- IP Professional
- Industry Leading Data Capabilities
- Powerful AI technology
- Patent DNA Extraction
Browse by: Latest US Patents, China's latest patents, Technical Efficacy Thesaurus, Application Domain, Technology Topic.
© 2024 PatSnap. All rights reserved.Legal|Privacy policy|Modern Slavery Act Transparency Statement|Sitemap