Aerially-photographed vehicle real-time detection method based on deep learning
A real-time detection and deep learning technology, applied in the field of image recognition, can solve the problems of large feature scale, low utilization of aerial vehicle target information, insufficient training of difficult vehicle samples, etc., to achieve high information utilization and vehicle characteristics. Enrich and improve the effect of detection accuracy
- Summary
- Abstract
- Description
- Claims
- Application Information
AI Technical Summary
Problems solved by technology
Method used
Image
Examples
Embodiment Construction
[0033] The present invention will be described in detail below in conjunction with the accompanying drawings and specific embodiments.
[0034] refer to figure 1 , a method for real-time detection of aerial vehicles based on deep learning, comprising the following steps:
[0035] Step 1) Construct aerial vehicle dataset:
[0036] Step 1a) Extract a frame every 20 frames from the continuous frame images in the video of the road running vehicle taken by the drone, save it in the JPEGImages folder in the form of pictures, and name each picture with a different name, where The resolution of the video is 1920×1080, and the number of pictures saved in the JPEGImages folder is not less than 1000;
[0037] Step 1b) mark the different vehicle targets contained in each picture in the JPEGImages folder:
[0038] Step 1b1) Mark the category c and position coordinates (x1, y1, x2, y2) of the vehicle target, where the category c belongs to the six types of vehicle targets of cars, buses,...
PUM
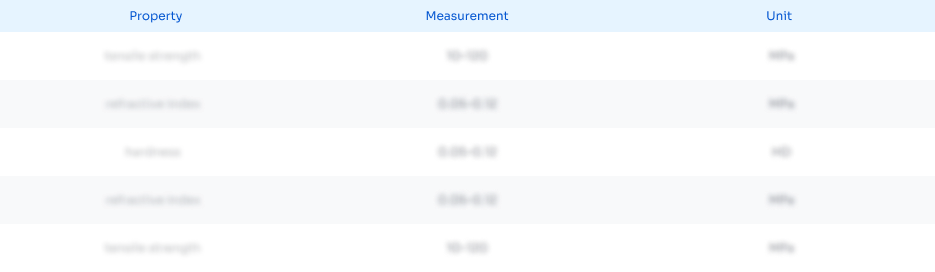
Abstract
Description
Claims
Application Information
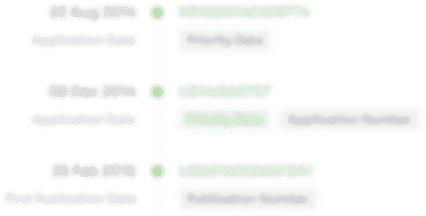
- R&D
- Intellectual Property
- Life Sciences
- Materials
- Tech Scout
- Unparalleled Data Quality
- Higher Quality Content
- 60% Fewer Hallucinations
Browse by: Latest US Patents, China's latest patents, Technical Efficacy Thesaurus, Application Domain, Technology Topic, Popular Technical Reports.
© 2025 PatSnap. All rights reserved.Legal|Privacy policy|Modern Slavery Act Transparency Statement|Sitemap|About US| Contact US: help@patsnap.com