Global normalized reader systems and methods
A normalized and global technology, applied in inference methods, neural learning methods, instruments, etc., can solve problems such as high cost, no system performance training data, and limited document applicability.
- Summary
- Abstract
- Description
- Claims
- Application Information
AI Technical Summary
Problems solved by technology
Method used
Image
Examples
Embodiment approach
[0036] Given a document and a question, extractive question answering can be thought of as a search problem. figure 1 A question answering method according to an embodiment of the disclosure is depicted. In an embodiment, the sentence containing the answer span is selected (105); then, the first word of the answer span is selected (110), and finally the last word is selected (115).
[0037] The process described in figure 2 The example shown in uses the actual model's predictions. figure 2 A Global Normalized Reader (GNR) model implementation for answering questions according to an embodiment of the disclosure is depicted. In the depicted embodiment, the probabilities are global, normalized over the bundle, rather than local probabilities. It should be noted that the final predicted probability is greater than the first decision and the second decision, and the model is more reliable during the search process.
[0038] Initially, the higher score was attributed to the pi...
PUM
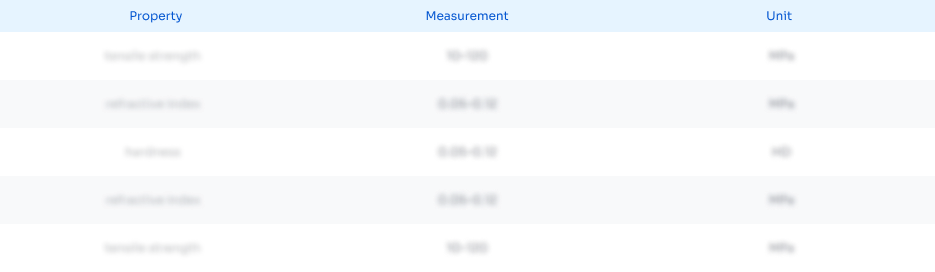
Abstract
Description
Claims
Application Information
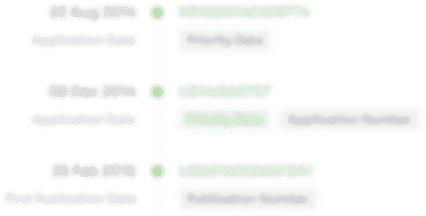
- R&D Engineer
- R&D Manager
- IP Professional
- Industry Leading Data Capabilities
- Powerful AI technology
- Patent DNA Extraction
Browse by: Latest US Patents, China's latest patents, Technical Efficacy Thesaurus, Application Domain, Technology Topic.
© 2024 PatSnap. All rights reserved.Legal|Privacy policy|Modern Slavery Act Transparency Statement|Sitemap