Traffic image semantic segmentation method based on multi-feature map
A semantic segmentation, multi-feature technology, applied in the field of computer vision and pattern recognition, can solve the problem of low accuracy of semantic segmentation, and achieve the effect of improving accuracy
- Summary
- Abstract
- Description
- Claims
- Application Information
AI Technical Summary
Problems solved by technology
Method used
Image
Examples
Embodiment Construction
[0046] The specific embodiments of the present invention will be described in detail below in conjunction with the technical solutions and accompanying drawings.
[0047] Such as figure 1 As shown, a traffic image semantic segmentation method based on multi-feature maps includes the following steps:
[0048] A. Obtain multi-feature map training samples
[0049] A1. Get if figure 2 The disparity map shown;
[0050] A2. Get if image 3 The heightmap shown;
[0051] A3. Get if Figure 4 The angle diagram shown;
[0052] B. Construct a network model and train the constructed network model
[0053] B1, build as Figure 5 The network model shown;
[0054] The input of the network model is a feature map and a color map, and the feature map includes a disparity map, a height map, and an angle map. The input of the network model is disparity map, height map, angle map and color map from left to right. The network model consists of an encoder network and a decoder network. Th...
PUM
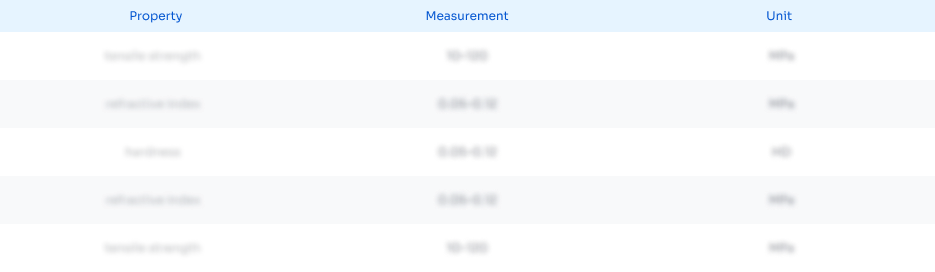
Abstract
Description
Claims
Application Information
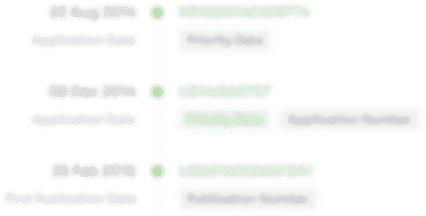
- R&D Engineer
- R&D Manager
- IP Professional
- Industry Leading Data Capabilities
- Powerful AI technology
- Patent DNA Extraction
Browse by: Latest US Patents, China's latest patents, Technical Efficacy Thesaurus, Application Domain, Technology Topic, Popular Technical Reports.
© 2024 PatSnap. All rights reserved.Legal|Privacy policy|Modern Slavery Act Transparency Statement|Sitemap|About US| Contact US: help@patsnap.com