Weighted convolutional autoencoder-long short-term memory network-based crowd anomaly detection method
A long-short-term memory, convolutional self-encoding technology, applied in the field of computer vision, can solve the problems of reconstructing normal behavior, model influence, difficult training of GAN models, etc., to achieve the effect of accurate detection and expansion of reconstruction errors
- Summary
- Abstract
- Description
- Claims
- Application Information
AI Technical Summary
Problems solved by technology
Method used
Image
Examples
Embodiment Construction
[0035] The present invention will be further described below in conjunction with the accompanying drawings, but the protection scope of the present invention is not limited thereto.
[0036] figure 1 The system flow chart of crowd anomaly detection based on weighted convolutional self-encoded long-short-term memory network is given:
[0037] The crowd anomaly detection method proposed by the present invention detects the foreground information in the original data and the corresponding optical flow information, and suppresses background noise interference. By establishing a WCAE-LSTM network, the spatial change information is obtained by convolutional self-encoding, and the temporal change information is obtained by three convolutional long-term short-term memory network modules, and the original data and its corresponding optical flow information are reconstructed. Based on the reconstruction Anomaly detection for errors. In addition, through the global detection of abnorma...
PUM
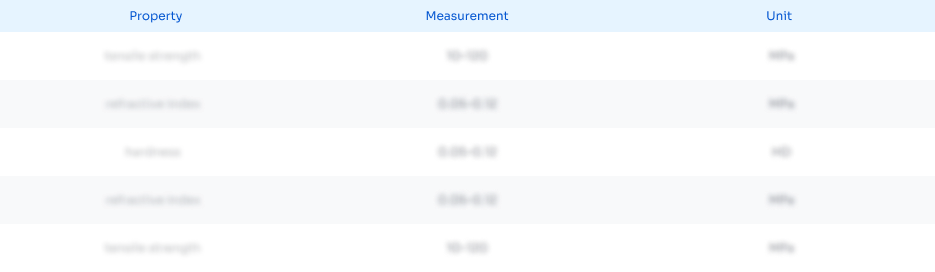
Abstract
Description
Claims
Application Information
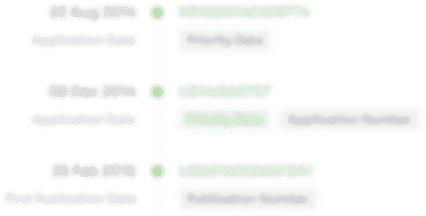
- R&D Engineer
- R&D Manager
- IP Professional
- Industry Leading Data Capabilities
- Powerful AI technology
- Patent DNA Extraction
Browse by: Latest US Patents, China's latest patents, Technical Efficacy Thesaurus, Application Domain, Technology Topic, Popular Technical Reports.
© 2024 PatSnap. All rights reserved.Legal|Privacy policy|Modern Slavery Act Transparency Statement|Sitemap|About US| Contact US: help@patsnap.com