Gene classification method and system based on clustering and random forest algorithms
A technology of random forest algorithm and classification method, applied in the field of gene classification, can solve problems such as inaccurate classification, and achieve the effect of improving clustering accuracy, reducing complexity, and accurately dividing
- Summary
- Abstract
- Description
- Claims
- Application Information
AI Technical Summary
Problems solved by technology
Method used
Image
Examples
Embodiment Construction
[0059] The specific embodiments of the present invention will be further described below in conjunction with the accompanying drawings.
[0060] An embodiment of the gene classification method based on the clustering and random forest algorithm of the present invention.
[0061] The random forest algorithm has a relatively large dependence on the training set. The number of elements in the training sample set is large and the samples are rich, so the model will be trained more perfectly. However, for small sample genetic data, the training set has fewer elements, and the random forest model Accuracy is not guaranteed. Therefore, gene classification method of the present invention has fused clustering algorithm and random forest algorithm, as figure 2 As shown in , the cluster centers obtained by the clustering algorithm are added to the training set as an expansion of the training set to fully train the random forest model and obtain better classification results. The flow ...
PUM
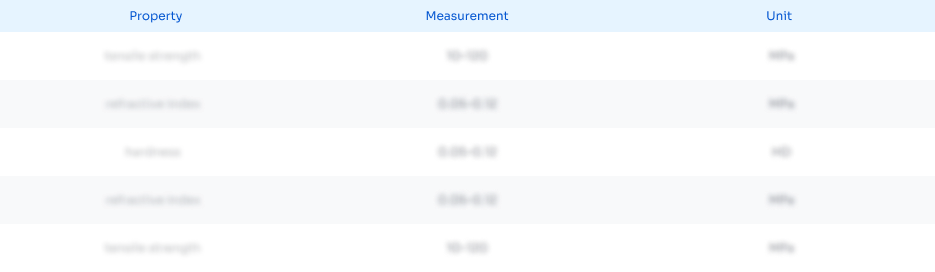
Abstract
Description
Claims
Application Information
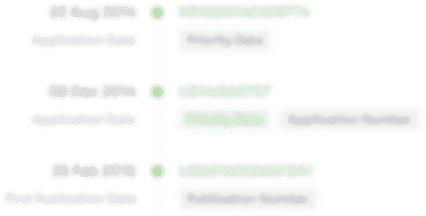
- R&D Engineer
- R&D Manager
- IP Professional
- Industry Leading Data Capabilities
- Powerful AI technology
- Patent DNA Extraction
Browse by: Latest US Patents, China's latest patents, Technical Efficacy Thesaurus, Application Domain, Technology Topic.
© 2024 PatSnap. All rights reserved.Legal|Privacy policy|Modern Slavery Act Transparency Statement|Sitemap