Polarized SAR Classification Method Based on Deep Bidirectional LSTM Siamese Network
A technology of twin network and classification method, applied in the field of polarimetric SAR classification based on deep convolutional bidirectional LSTM twin network, can solve the problems of high professional knowledge requirement of polarimetric SAR, complex manual design and extraction, unreasonable utilization methods, etc. , to overcome the problem of a large number of labeled sample information, reduce manual labeling work, and reduce complex and high-cost effects
- Summary
- Abstract
- Description
- Claims
- Application Information
AI Technical Summary
Problems solved by technology
Method used
Image
Examples
Embodiment Construction
[0033] In order to make the object, technical solution and advantages of the present invention clearer, the present invention will be introduced and described in detail below in conjunction with the accompanying drawings and specific embodiments.
[0034] Refer to attached figure 1 , the specific implementation steps of the present invention are as follows:
[0035] Step 1. Input a polarimetric SAR image to be classified with a size of 300*270 and the real object label information corresponding to the polarimetric SAR image.
[0036] Step 2. Filter the polarimetric SAR image data to be classified by using the Lee filtering method to remove coherent speckle noise interference, and obtain the filtered polarimetric SAR image data to be classified.
[0037] Step 3. Extract the polarization feature vector of each pixel from the polarization covariance matrix C of the polarimetric SAR image data to be classified after filtering, and use the spatial neighborhood information to obtai...
PUM
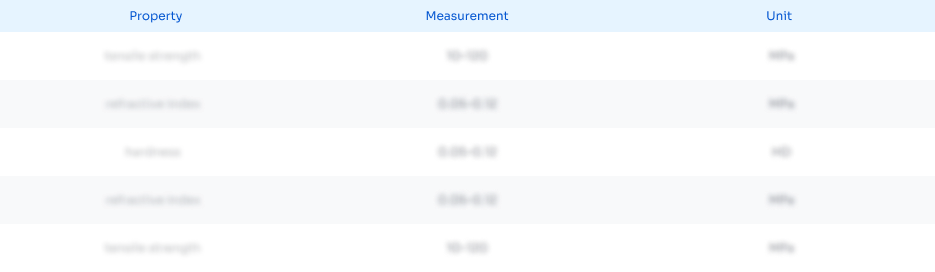
Abstract
Description
Claims
Application Information
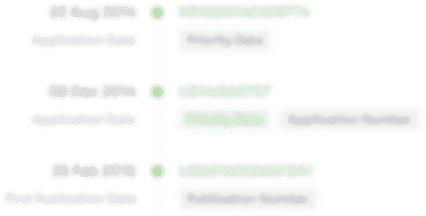
- Generate Ideas
- Intellectual Property
- Life Sciences
- Materials
- Tech Scout
- Unparalleled Data Quality
- Higher Quality Content
- 60% Fewer Hallucinations
Browse by: Latest US Patents, China's latest patents, Technical Efficacy Thesaurus, Application Domain, Technology Topic, Popular Technical Reports.
© 2025 PatSnap. All rights reserved.Legal|Privacy policy|Modern Slavery Act Transparency Statement|Sitemap|About US| Contact US: help@patsnap.com