Unbalanced data set-oriented extreme learning machine based transformer fault diagnosis method
An extreme learning machine, transformer fault technology, applied in the direction of instruments, computer parts, character and pattern recognition, etc., can solve the problems of low recognition rate of a few fault sets, limited amount of information, and difficulty in determining the data distribution of a few fault sets.
- Summary
- Abstract
- Description
- Claims
- Application Information
AI Technical Summary
Problems solved by technology
Method used
Image
Examples
Embodiment Construction
[0054] The present invention will be described in detail below in conjunction with the accompanying drawings and specific embodiments.
[0055] The present invention provides an extreme learning machine transformer fault diagnosis method for unbalanced data sets, the process of which is as follows figure 1 As shown, the specific steps are as follows:
[0056] Step 1: Data processing stage,
[0057] Step 1.1: Collect the unbalanced sample set S={(x 1 ,t 1 ),(x 2 ,t 2 )...(x n ,t n )} is divided into training samples and test samples according to the ratio of 6:1; among them, x i Represents sample attributes, i=1, 2, 3, 4, 5, 6, specifically including hydrogen, methane, ethane, ethylene, acetylene, carbon monoxide, six attributes; t i Represents the category label, i=1, 2, 3, 4, 5, 6, 1, 2, 3, 4, 5, 6 respectively correspond to normal state, medium temperature overheating, high temperature overheating, partial discharge, spark discharge, arc discharge, and use The PAM a...
PUM
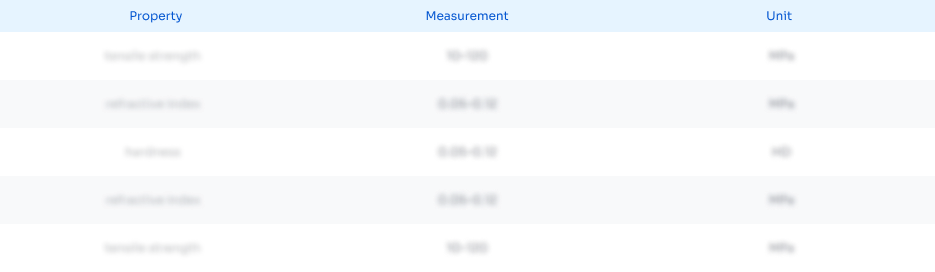
Abstract
Description
Claims
Application Information
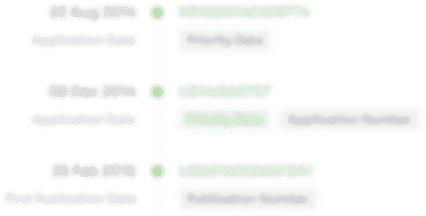
- R&D Engineer
- R&D Manager
- IP Professional
- Industry Leading Data Capabilities
- Powerful AI technology
- Patent DNA Extraction
Browse by: Latest US Patents, China's latest patents, Technical Efficacy Thesaurus, Application Domain, Technology Topic, Popular Technical Reports.
© 2024 PatSnap. All rights reserved.Legal|Privacy policy|Modern Slavery Act Transparency Statement|Sitemap|About US| Contact US: help@patsnap.com