Deep-learning-based SDN (Software Defined network) flow forecasting method
A technology of network traffic and deep learning, applied in the direction of data exchange network, digital transmission system, electrical components, etc., to achieve the effect of reasonable optimization of network design, improvement of operating efficiency, and load balancing
- Summary
- Abstract
- Description
- Claims
- Application Information
AI Technical Summary
Problems solved by technology
Method used
Image
Examples
Embodiment Construction
[0028] The present invention will be further described below in conjunction with the accompanying drawings.
[0029] The present invention provides a deep learning-based SDN network traffic prediction method, which provides a traffic prediction function for the unbalanced distribution of SDN network traffic, obtains the traffic of the forwarding layer, and performs prediction and analysis at the control layer to realize the overall optimization of the application layer. The network traffic prediction strategy runs through the entire system to ensure the stable and efficient operation of the network and improve the service quality of the system.
[0030] Step 1: Build an SDN network traffic prediction model, and add corresponding modules to each layer of SDN, such as figure 1 Shown:
[0031] The application layer includes an application service management module and an application regulation management module;
[0032] The control layer includes a traffic prediction analysis ...
PUM
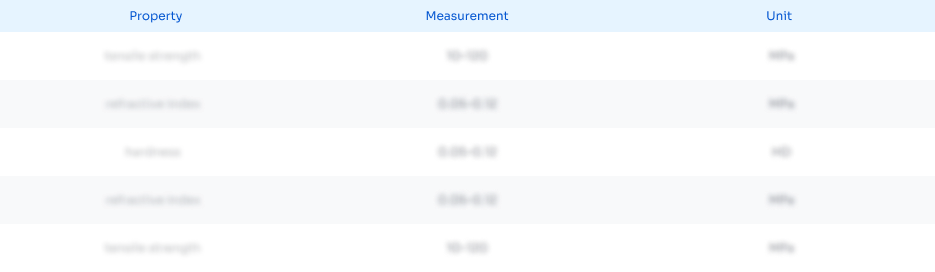
Abstract
Description
Claims
Application Information
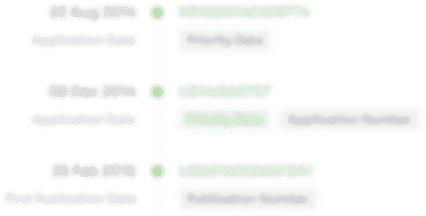
- Generate Ideas
- Intellectual Property
- Life Sciences
- Materials
- Tech Scout
- Unparalleled Data Quality
- Higher Quality Content
- 60% Fewer Hallucinations
Browse by: Latest US Patents, China's latest patents, Technical Efficacy Thesaurus, Application Domain, Technology Topic, Popular Technical Reports.
© 2025 PatSnap. All rights reserved.Legal|Privacy policy|Modern Slavery Act Transparency Statement|Sitemap|About US| Contact US: help@patsnap.com