A Width Learning Method Based on Principal Component Analysis
A principal component analysis and width technology, applied in neural learning methods, instruments, biological neural network models, etc., can solve the problems of parameter adjustment uncertainty, long training time, and large amount of calculation, so as to facilitate real-time update and ensure recognition. Accuracy, the effect of shortening training time
- Summary
- Abstract
- Description
- Claims
- Application Information
AI Technical Summary
Problems solved by technology
Method used
Image
Examples
Embodiment Construction
[0060] The following is attached figure 1 , and specific embodiments, the present invention is further described.
[0061] A width learning method based on principal component analysis, comprising: a node setting step of the width learning network, a weight parameter setting step of the width learning network, a parameter training step of the width learning network, and a recognition learning step of the width learning network;
[0062] The node setting step of the width learning network is based on the principal component analysis method, and the obtained principal component is used as the characteristic node of the width learning network, and all the characteristic nodes obtained based on the principal component analysis step are linearly combined to obtain several enhanced nodes;
[0063] The weight parameter setting step of the width learning network is to use the feature nodes and the enhancement nodes of the width learning network as the input layer of the width learning...
PUM
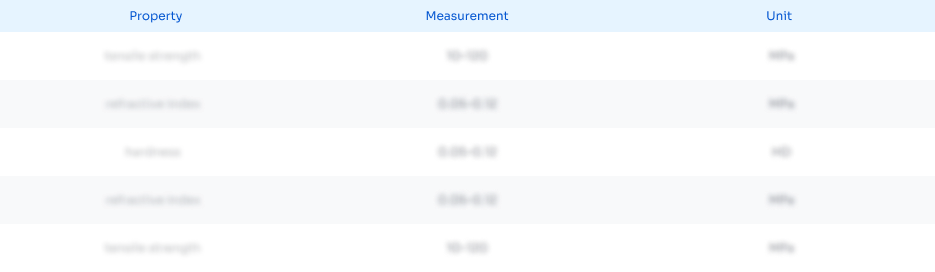
Abstract
Description
Claims
Application Information
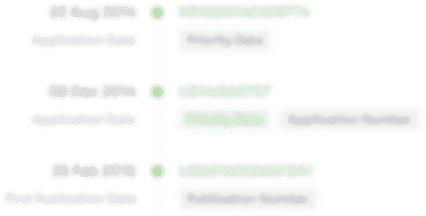
- R&D
- Intellectual Property
- Life Sciences
- Materials
- Tech Scout
- Unparalleled Data Quality
- Higher Quality Content
- 60% Fewer Hallucinations
Browse by: Latest US Patents, China's latest patents, Technical Efficacy Thesaurus, Application Domain, Technology Topic, Popular Technical Reports.
© 2025 PatSnap. All rights reserved.Legal|Privacy policy|Modern Slavery Act Transparency Statement|Sitemap|About US| Contact US: help@patsnap.com