Semantic segmentation of weakly supervised images based on iterative mining of common features of objects
A technology of semantic segmentation and common features, applied in the field of pattern recognition, can solve the problems of increased difficulty in neural network design and training, achieve reliable semantic segmentation, reduce application costs, and have a wide range of application prospects
- Summary
- Abstract
- Description
- Claims
- Application Information
AI Technical Summary
Problems solved by technology
Method used
Image
Examples
Embodiment Construction
[0051] The weakly supervised image semantic segmentation method based on iteratively mining the common features of objects proposed by the present invention will be further described in detail below in conjunction with the accompanying drawings and specific embodiments. The following examples are only used to illustrate the present invention, but are not intended to limit the scope of the present invention.
[0052] The weakly supervised image semantic segmentation method based on the iterative mining object common feature proposed by the present invention is divided into a training stage and a use stage, including the following steps:
[0053] 1) Training phase; the overall process is as follows figure 1 As shown, the specific steps are as follows:
[0054] 1-1) Obtain a training data set;
[0055] The training data set includes training images and category labels corresponding to the images. When constructing the training data set, it is first necessary to define the obje...
PUM
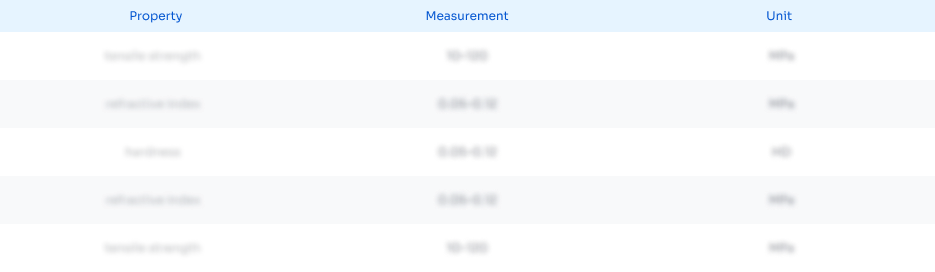
Abstract
Description
Claims
Application Information
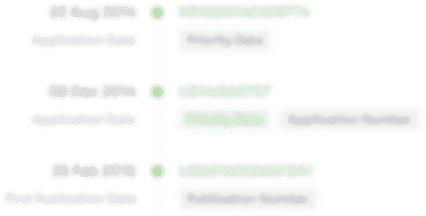
- R&D Engineer
- R&D Manager
- IP Professional
- Industry Leading Data Capabilities
- Powerful AI technology
- Patent DNA Extraction
Browse by: Latest US Patents, China's latest patents, Technical Efficacy Thesaurus, Application Domain, Technology Topic, Popular Technical Reports.
© 2024 PatSnap. All rights reserved.Legal|Privacy policy|Modern Slavery Act Transparency Statement|Sitemap|About US| Contact US: help@patsnap.com