An image segmentation method based on improved intuitionistic fuzzy C-means clustering
A fuzzy intuition and mean value clustering technology, applied in character and pattern recognition, instruments, computer components, etc., can solve problems such as inaccurate segmentation results, large clustering errors, and ignoring spatial correlation of pixels
- Summary
- Abstract
- Description
- Claims
- Application Information
AI Technical Summary
Problems solved by technology
Method used
Image
Examples
Embodiment 1
[0137] Example 1: Segmentation of simple square images
[0138] In order to verify the effectiveness of the method of the present invention for different types of images, first use the method of the present invention and six methods of FCM, IFCM, KIFCM, IFCM-S, and IIFCM to segment a simple-structured block image with a size of 256*256. The simulated square image consists of four parts with different gray values of 7, 78, 214 and 251 (as shown in Fig. 2(a)). The classes in the image are divided into patches of different sizes. For simplicity, call them C1 (gray value 7), C2 (gray value 78), C3 (gray value 214), and C4 (gray value 251). Figure 2(c) is the ground-truth segmentation result map of the square image, which is divided into four parts, corresponding to four categories. To verify the robustness of different methods to noise, images polluted by 1% salt and pepper noise (S&P 1%) (as shown in Fig. 2(b)) are processed by the above six methods.
[0139] The experimenta...
Embodiment 2
[0153] Example 2: Segmentation of MRI brain images
[0154] The human brain has a complex structure. Noise and blurring between different tissues make segmentation of MRI brain images difficult. Segmentation requires precise segmentation of the three main tissues of the brain: cerebrospinal fluid (CSF), gray matter (GM) and white matter (WM). In this embodiment, segmentation experiments are performed on MRI brain images in order to further compare the performance of different methods. The simulated MRI brain images and ground-truth segmentation results used in the experiment can be obtained from Brain Web, the publicly available dataset Simulated Brain Dataset (SBD). Keeping the same parameter configuration as the block image experiment, different methods are validated. It should be noted that the number of categories to be clustered is c=4, corresponding to GM, WM, CSF and background respectively.
[0155] Figure 3(a) is a simulated MRI brain image with a size of 217*181....
PUM
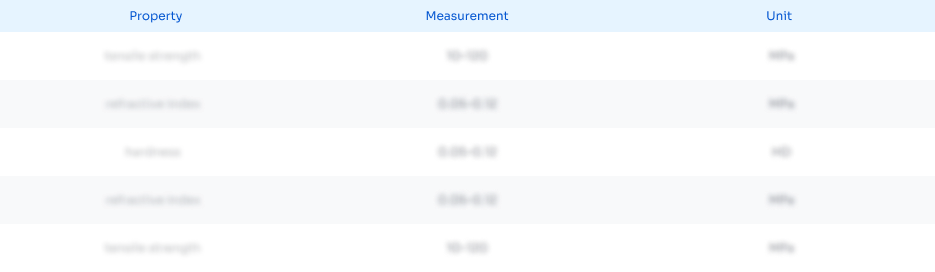
Abstract
Description
Claims
Application Information
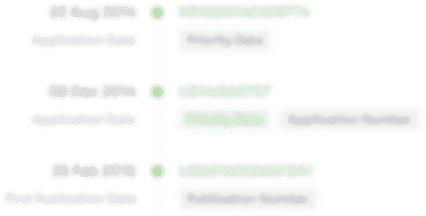
- R&D Engineer
- R&D Manager
- IP Professional
- Industry Leading Data Capabilities
- Powerful AI technology
- Patent DNA Extraction
Browse by: Latest US Patents, China's latest patents, Technical Efficacy Thesaurus, Application Domain, Technology Topic, Popular Technical Reports.
© 2024 PatSnap. All rights reserved.Legal|Privacy policy|Modern Slavery Act Transparency Statement|Sitemap|About US| Contact US: help@patsnap.com