A small object detection method in real scene based on multi-task generation antagonistic network
A detection method and real scene technology, applied in the field of computer vision and object detection, can solve the problems of small objects cannot be clearly identified, poor adaptive ability, etc.
- Summary
- Abstract
- Description
- Claims
- Application Information
AI Technical Summary
Problems solved by technology
Method used
Image
Examples
Embodiment 1
[0060] This embodiment proposes a real-scene small object detection method based on a multi-task generative confrontation network, such as Figure 4 As shown, the detection method includes:
[0061] Step 1: determine a training sample set, and use the training sample set to train an object detector; the training sample set contains a plurality of images;
[0062] Step 2: using the object detector to intercept and generate training samples for the multi-task generation confrontation network;
[0063] Step 3: constructing a multi-task generative adversarial network training sample according to the training sample; the multi-task generative adversarial network training sample includes a positive training sample and a negative training sample;
[0064] Step 4: taking the training sample generated by interception as a high-resolution image, and using bilinear interpolation to downsample the high-resolution image by 4 times to obtain an image as a corresponding low-resolution image...
Embodiment 2
[0072] This embodiment is a further limitation of the method for detecting small objects in real scenes described in Embodiment 1. The training sample set can be collected by itself according to actual needs, and then a corresponding object category database can be constructed, or an existing public object detection database can be selected. Such as Pascal VOC, Microsoft COCO and other databases. In order to facilitate comparison with other existing methods, this embodiment uses the Microsoft COCO data set as the training sample set, and uses the 115K data in the Microsoft COCO data set as the training set sample, and uses the 5K data in the Microsoft COCO data set as the verification set sample, Use the 5K data in the Microsoft COCO dataset as a test set sample;
[0073] The object images in the training samples are divided into three grades of large, medium and small according to the size of the area, wherein a larger object image refers to an image with an area larger than ...
Embodiment 3
[0076] This embodiment is a further limitation of the method for detecting small objects in real scenes described in Embodiment 1 or 2. The specific process for the object detector in step 2 to intercept and generate training samples for a multi-task generation adversarial network includes:
[0077] The first step: using the object detector to predict object position information for each image in the training sample set;
[0078] Step 2: Intercept 600 images of areas most likely to contain objects in each image after object position information prediction;
[0079] The third step: saving the intercepted image area, and the saved area image is used as a training sample of the multi-task generation confrontation network.
[0080] In this embodiment, the object detector is used to predict the object position information of each image in the Microsoft COCO training sample set, and 600 regions most likely to contain objects are intercepted from each image and saved, and these saved...
PUM
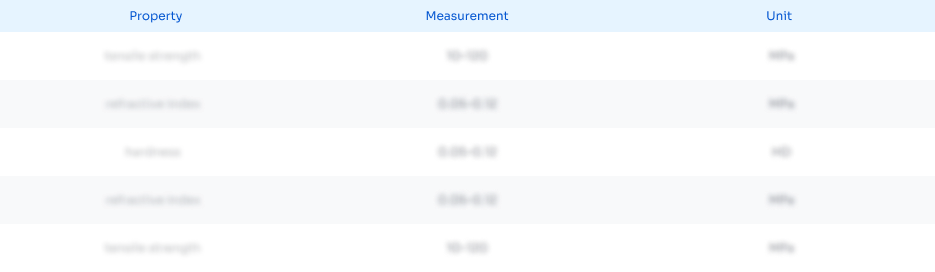
Abstract
Description
Claims
Application Information
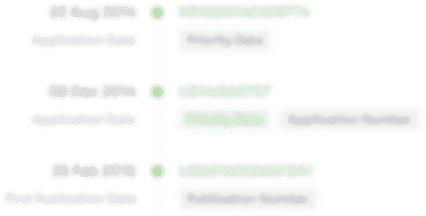
- R&D Engineer
- R&D Manager
- IP Professional
- Industry Leading Data Capabilities
- Powerful AI technology
- Patent DNA Extraction
Browse by: Latest US Patents, China's latest patents, Technical Efficacy Thesaurus, Application Domain, Technology Topic, Popular Technical Reports.
© 2024 PatSnap. All rights reserved.Legal|Privacy policy|Modern Slavery Act Transparency Statement|Sitemap|About US| Contact US: help@patsnap.com