A high-speed real-time quantization structure and operation implementation method for deep neural network
A technology of deep neural network and implementation method, which is applied in the direction of neural learning method, biological neural network model, physical realization, etc., and can solve problems such as inaccuracy
- Summary
- Abstract
- Description
- Claims
- Application Information
AI Technical Summary
Problems solved by technology
Method used
Image
Examples
Embodiment 1
[0069] The deep neural network can be applied in the image recognition of image processing. The deep neural network is composed of a multi-layer network. Here is an example of the result of one layer and the image operation. The input data is the gray value of the image, as shown in Table 3, Table 3 It is a binary value, and the value corresponds to the gray value of the image. The deep neural network completes the convolution and other operations on the image, and recognizes and classifies the image according to the calculation results.
[0070] deep neural network
[0071] Such as figure 1 As shown, the expression of parameters in the form of integer multiple powers of parameters can be expressed in a unit (same layer) with relatively concentrated operations, that is, as long as the relative relationship of parameters in the unit is in the form of integer multiple powers, a shared weight, you can use parameters in the form of powers of integer multiples. parameters such a...
Embodiment 2
[0085] The same applies to image recognition. The unquantified raw data of the deep network are shown in Table 5.
[0086] The expression of parameters in the form of integer multiple powers of parameters can be expressed in a unit (same layer) with relatively concentrated operations, that is, as long as the relative relationship of the parameters in the unit is the parameters in the form of integer multiple powers, shared weights are proposed. You can use parameters in the form of powers of integer multiples. The parameters shown in the table are temporarily quantified by using the 4th power of 2 as the largest value in the corresponding parameter, and the 4th power of 2 corresponds to 6.84, the 3rd power of 2 corresponds to 3.42, the 2nd power of 2 corresponds to 1.71, and the 1st power of 2 corresponds to 6.84. The power of 2 corresponds to 0.855, the 0th power of 2 corresponds to 0.4275, and the common coefficient 0.4275 is proposed. The quantified results are shown in T...
PUM
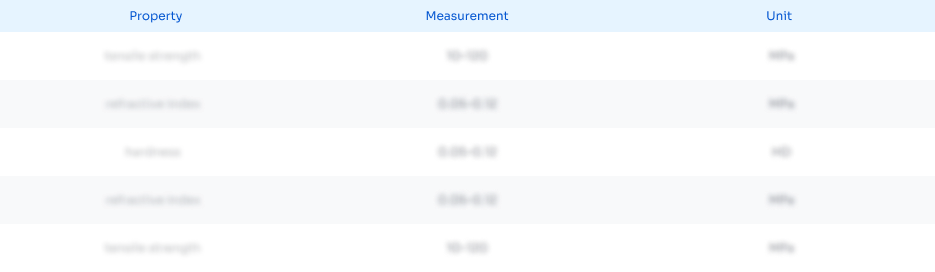
Abstract
Description
Claims
Application Information
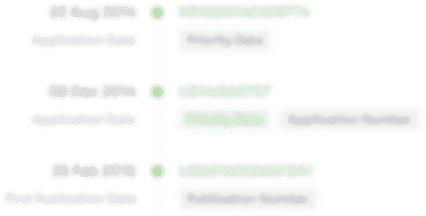
- R&D
- Intellectual Property
- Life Sciences
- Materials
- Tech Scout
- Unparalleled Data Quality
- Higher Quality Content
- 60% Fewer Hallucinations
Browse by: Latest US Patents, China's latest patents, Technical Efficacy Thesaurus, Application Domain, Technology Topic, Popular Technical Reports.
© 2025 PatSnap. All rights reserved.Legal|Privacy policy|Modern Slavery Act Transparency Statement|Sitemap|About US| Contact US: help@patsnap.com