Multi-modal data analysis method and system based on high Laplacian regularization and low-rank representation
A low-rank representation and data analysis technology, applied in character and pattern recognition, medical automated diagnosis, instruments, etc.
- Summary
- Abstract
- Description
- Claims
- Application Information
AI Technical Summary
Problems solved by technology
Method used
Image
Examples
Embodiment 1
[0062] as attached figure 1 As shown, the multimodal data classification method of high Laplacian regularization and low rank representation of the present invention combines low rank representation and Laplacian regularization term to construct non-negative sparse super Laplacian regularization low rank representation model, and apply the non-negative sparse super-Laplacian regularized low-rank representation model to multimodal data analysis, including the following steps:
[0063] Step S100, data preprocessing: converting the multimodal data into two-dimensional matrix data, the row data in the matrix data represent samples, and the column data in the matrix data represent features;
[0064] Step S200, data grouping: based on the modal characteristics of multimodal data that can generate new modalities by superimposing the modalities, group the above-mentioned multiple matrix data to obtain multiple data matrices, each data matrix corresponds to a new modal state;
[0065...
Embodiment 2
[0102] In this embodiment, the multimodal data analysis system of high Laplacian regularization and low-rank representation includes:
[0103] The data processing module is used to perform data processing on multimodal data to obtain multiple data matrices, each data matrix corresponds to a modality, and the data in each data matrix is two-dimensional matrix data;
[0104] The data analysis module is used to combine the low-rank representation and the graph Laplacian regularization term to construct a non-negative sparse super-Laplacian regularization low-rank representation model, and through the non-negative sparse super-Laplacian regularization term Learn each data matrix to obtain a high-order Laplacian low-rank subspace;
[0105] A classification module for learning based on high-order Laplacian low-rank subspaces and support vector machines to obtain multiple classifiers;
[0106] The voting module is used to vote for the above multiple classifiers to obtain the final...
PUM
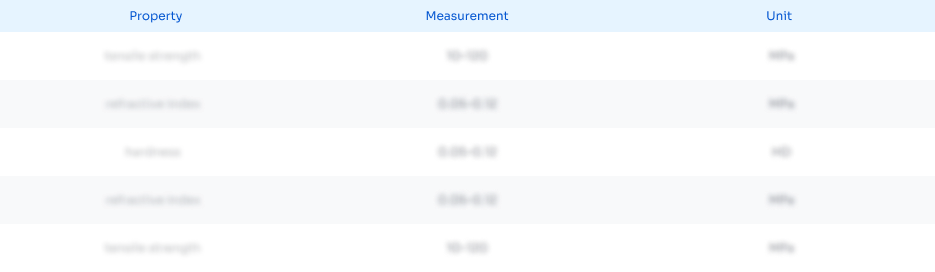
Abstract
Description
Claims
Application Information
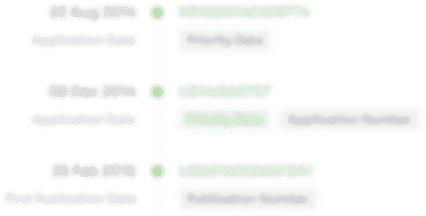
- R&D Engineer
- R&D Manager
- IP Professional
- Industry Leading Data Capabilities
- Powerful AI technology
- Patent DNA Extraction
Browse by: Latest US Patents, China's latest patents, Technical Efficacy Thesaurus, Application Domain, Technology Topic, Popular Technical Reports.
© 2024 PatSnap. All rights reserved.Legal|Privacy policy|Modern Slavery Act Transparency Statement|Sitemap|About US| Contact US: help@patsnap.com