A method for decomposing decorrelated multi-frequency empirical modes
An empirical mode decomposition, multi-frequency technology, applied in character and pattern recognition, pattern recognition in signals, instruments, etc., can solve the problems of time-frequency distribution confusion, affecting feature accuracy, lack of physical meaning of IMF components, etc. The effect of reducing the modal aliasing phenomenon, improving the feature extraction accuracy, and suppressing the modal aliasing phenomenon
- Summary
- Abstract
- Description
- Claims
- Application Information
AI Technical Summary
Problems solved by technology
Method used
Image
Examples
Embodiment 1
[0018] Such as Figure 1-2 As shown, a decorrelation multi-frequency empirical mode decomposition method includes the following steps:
[0019] S1: Use EMD to decompose the initial signal to obtain the first initial signal component PIMF1;
[0020] S2: use the PIMF1 component obtained in step S1 to obtain the frequency of the masking signal, and then construct the masking signal;
[0021] S3: Remove the masking signal obtained in step S2 from the initial signal to obtain a new signal, and then use EMD to decompose the new signal to obtain the first-order unmasked signal component MIMF1, and repeat steps S1 to S3 to obtain the second-order unmasking signal component MIMF2;
[0022] S4: Utilize the first two order unmasked signal components MIMF1 and MIMF2 obtained in step S3, and embed correlation coefficient processing to obtain the optimal intrinsic mode function, denoted as OIMF1;
[0023] S5: Remove the optimal OIMF1 from the initial signal, repeat steps S1 to S5 until t...
PUM
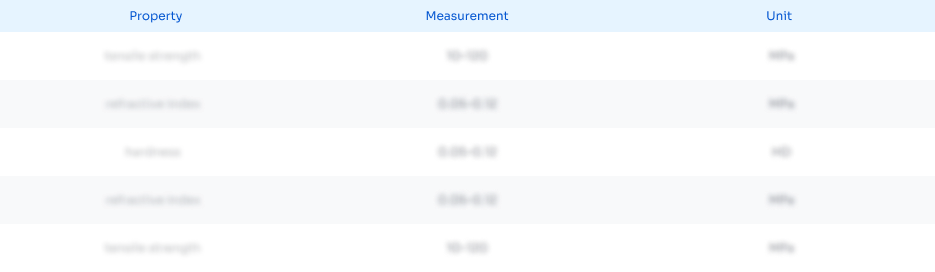
Abstract
Description
Claims
Application Information
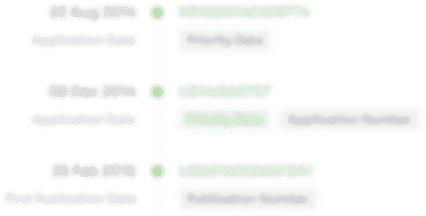
- R&D
- Intellectual Property
- Life Sciences
- Materials
- Tech Scout
- Unparalleled Data Quality
- Higher Quality Content
- 60% Fewer Hallucinations
Browse by: Latest US Patents, China's latest patents, Technical Efficacy Thesaurus, Application Domain, Technology Topic, Popular Technical Reports.
© 2025 PatSnap. All rights reserved.Legal|Privacy policy|Modern Slavery Act Transparency Statement|Sitemap|About US| Contact US: help@patsnap.com