A migration sparse coding image classification method based on dictionary domain adaptation
A sparse coding and classification method technology, applied in the field of machine learning, can solve problems such as differences in coding features and affecting classification performance, and achieve the effects of improving transferability, solving classification performance degradation, and improving knowledge transfer efficiency
- Summary
- Abstract
- Description
- Claims
- Application Information
AI Technical Summary
Problems solved by technology
Method used
Image
Examples
Embodiment 1
[0071] In order to reflect the credibility and classification performance of the algorithm, three types of benchmark datasets were selected in the experiment: USPS+MNIST handwritten digit dataset, COIL20 object recognition dataset and Office+Caltech256 object recognition dataset, and a total of 16 sets of cross-domain classification tasks were constructed. . The statistical information of each data set is shown in Table 1, and the images of some data sets are shown in figure 1 shown.
[0072] Table 1 Explanation of the experimental image dataset
[0073] dataset name
PUM
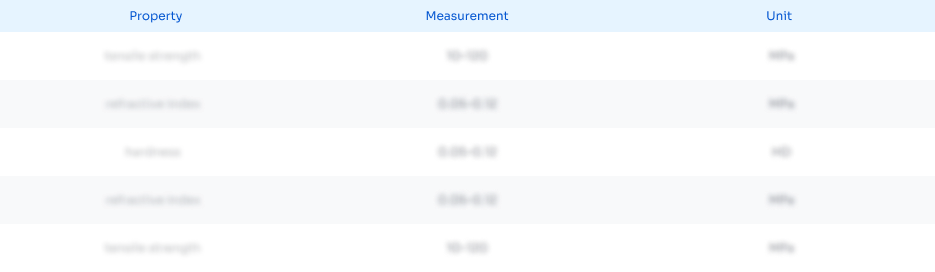
Abstract
Description
Claims
Application Information
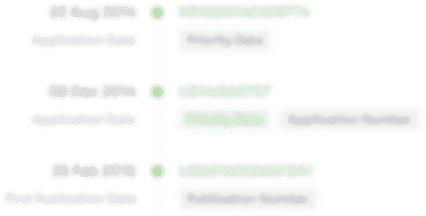
- R&D Engineer
- R&D Manager
- IP Professional
- Industry Leading Data Capabilities
- Powerful AI technology
- Patent DNA Extraction
Browse by: Latest US Patents, China's latest patents, Technical Efficacy Thesaurus, Application Domain, Technology Topic.
© 2024 PatSnap. All rights reserved.Legal|Privacy policy|Modern Slavery Act Transparency Statement|Sitemap