A binocular disparity estimation method based on cascaded geometric context neural network
A neural network, binocular disparity technology, applied in the field of computer vision, can solve the problems of target occlusion, difficult to find, low texture, etc., to achieve the effect of improving prediction accuracy and disparity estimation accuracy
- Summary
- Abstract
- Description
- Claims
- Application Information
AI Technical Summary
Problems solved by technology
Method used
Image
Examples
Embodiment Construction
[0034] A binocular parallax estimation method based on a cascaded geometric context neural network, comprising the following steps:
[0035] Step (1) Image preprocessing. Normalize the left and right images of the binocular image pair with the reference actual reference image, so that the image pixel values are in [-1,1];
[0036] Step (2) Construct the cascaded convolutional neural network CGCNet. Includes the following network layers:
[0037] 2-1. Construct a rough disparity image estimation layer. The network layer is mainly composed of GCNet (Geometry and ContextNetwork) network.
[0038] 2-2. Construct a parallax refinement layer. The network layer is RefineNet, and the rough disparity map generated in steps 2-3 is input to the network layer, and the output result is an accurate disparity map.
[0039] Construct a cascaded convolutional neural network CGCNet. Includes the following network layers:
[0040] 2-1. The GCnet network mainly combines two-dimensional a...
PUM
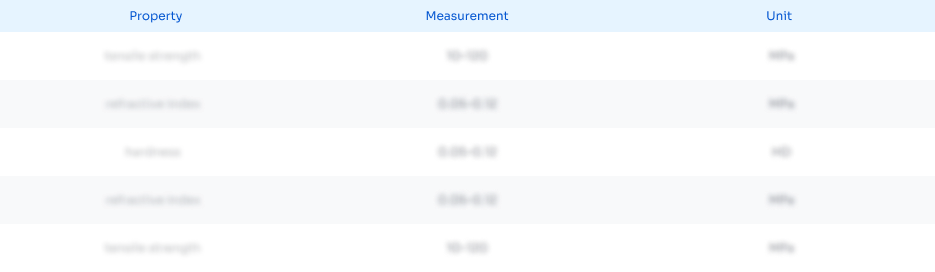
Abstract
Description
Claims
Application Information
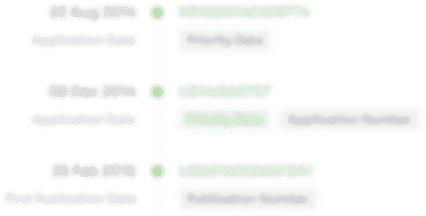
- R&D Engineer
- R&D Manager
- IP Professional
- Industry Leading Data Capabilities
- Powerful AI technology
- Patent DNA Extraction
Browse by: Latest US Patents, China's latest patents, Technical Efficacy Thesaurus, Application Domain, Technology Topic.
© 2024 PatSnap. All rights reserved.Legal|Privacy policy|Modern Slavery Act Transparency Statement|Sitemap