An efficient algorithm for detecting small defects in high resolution cloth images
A large-resolution, in-image technology, applied in image analysis, image enhancement, image data processing, etc., can solve the problems that the classification target does not occupy the total area of the image, cannot obtain category information, and has low accuracy, and achieves improved inspection. performance, suppressing invalid features, improving detection accuracy and speed
- Summary
- Abstract
- Description
- Claims
- Application Information
AI Technical Summary
Problems solved by technology
Method used
Image
Examples
Embodiment Construction
[0027] The present invention is further described below.
[0028] Implementation process of the present invention and embodiment are as follows:
[0029](1) Image collection. We use a camera with a resolution of 2560*1920 to shoot cloth images, and obtain a total of 5000 cloth images, and rename them as 1.jpg, 2.jpg, ..., 5000.jpg, and then Scale the picture to a size of 1024*768, and use the labelImg tool to label the captured image to obtain the label about the defect in the image. The label of the defect includes the coordinates (x1, y1) of the upper left corner of the defect in the image, the coordinates (x2, y2) of the lower right corner and the category defectN of the defect, where N ∈ {1, 2, 3, ..., 9}, means There are a total of 9 types of defects in the data set. The defects are oil stains, jumping flowers, missing warps, hanging warps, thin weaves, hair holes, scratch holes, hair spots, and prick holes. The order of defects corresponds to the number of N in defectN....
PUM
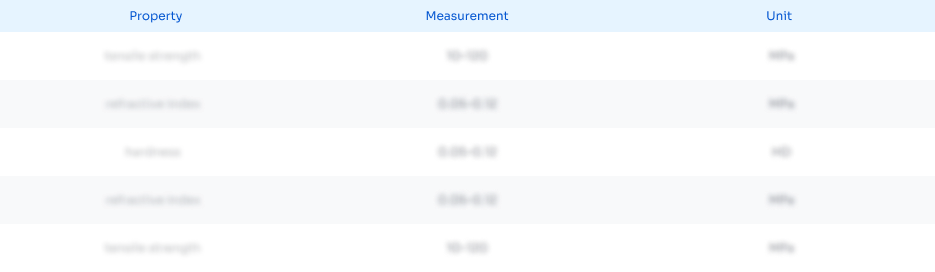
Abstract
Description
Claims
Application Information
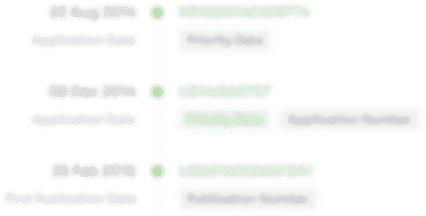
- R&D Engineer
- R&D Manager
- IP Professional
- Industry Leading Data Capabilities
- Powerful AI technology
- Patent DNA Extraction
Browse by: Latest US Patents, China's latest patents, Technical Efficacy Thesaurus, Application Domain, Technology Topic.
© 2024 PatSnap. All rights reserved.Legal|Privacy policy|Modern Slavery Act Transparency Statement|Sitemap