Bearing fault diagnosis based on pseudo-tag semi-supervised kernel local Fisher discriminant analysis
A Fisher discrimination and fault diagnosis technology, applied in the testing of computer parts, mechanical parts, character and pattern recognition, etc., can solve problems such as long-term local optimal solutions
- Summary
- Abstract
- Description
- Claims
- Application Information
AI Technical Summary
Problems solved by technology
Method used
Image
Examples
Embodiment 1
[0074] Based on pseudo-label semi-supervised nuclear local Fisher discriminant analysis for bearing fault diagnosis, the local Fisher discriminant analysis algorithm includes the following specific steps:
[0075] Let x i ∈R d Represents the i-th sample vector, and its corresponding class label is y i ∈{1,2,...,c}, c is the number of categories. Let X n ={x 1 , X 2 ,..., x i ,..., x n }∈R d×n Represents the labeled sample data matrix, X m ={x 1 , X 2 ,..., x i ,..., x m }∈R d×m Represents the overall sample data matrix, where m represents the number of training samples, n is the number of labeled samples, and m>n. Let X m ={X n , X u }, X u Is a collection of unlabeled samples. Suppose z i ∈R r (1≤r≤d) is through the matrix T ∈ R d×r The projection representation of the transformed low-dimensional subspace: z i = T T x i .
[0076] The local Fisher discriminant analysis algorithm (LFDA) can be expressed by the following optimization problem:
[0077]
[0078] Here, S lb , S lw ∈...
Embodiment 2
[0106] Based on pseudo-label semi-supervised nuclear local Fisher discriminant analysis for bearing fault diagnosis, the density peak clustering algorithm used for pseudo-label generation includes the following specific steps:
[0107] Given data set X m ={x 1 , X2,..., x i ,..., x m }∈R d×m , Where x i ∈R d Represents the i-th sample vector, for each sample point x i Firstly calculate its local density value ρ quantitatively i Distance δ from the sample point with higher distance density i , And their definitions are as follows:
[0108]
[0109] Here parameter d c To cut off distance need to be specified in advance, d ij Represents x i And x j Euclidean distance.
[0110] Further set Means A descending sequence of, which satisfies:
[0111]
[0112]
[0113] Obviously, it is not difficult to find from the above formula that for the sample points whose density value is the local or global maximum, their δ i Will be lower than the δ of other sample points j The value is much larger...
Embodiment 3
[0126] Based on the pseudo-label semi-supervised nuclear local Fisher discriminant analysis bearing fault diagnosis, the pseudo-label semi-supervised nuclear local Fisher discriminant analysis bearing fault diagnosis method includes the following specific steps:
[0127] Use the density peak clustering algorithm to analyze all sample sets X m Perform cluster analysis to get the cluster label set of sample points And whether it is an identification set of boundary points What needs to be explained here is the number of clusters n c It does not need to be the same as the number of categories, which makes it better to adapt to multi-modal data distribution. Construct local inter-cluster divergence S according to the above information ulb And local clustering divergence S ulw The regularization term is specifically expressed as follows:
[0128]
[0129]
[0130] Here W ulb , W ulw Is an m×m matrix, and
[0131]
[0132]
[0133] Represented in cluster c i ∈{1, 2,..., n c } The number...
PUM
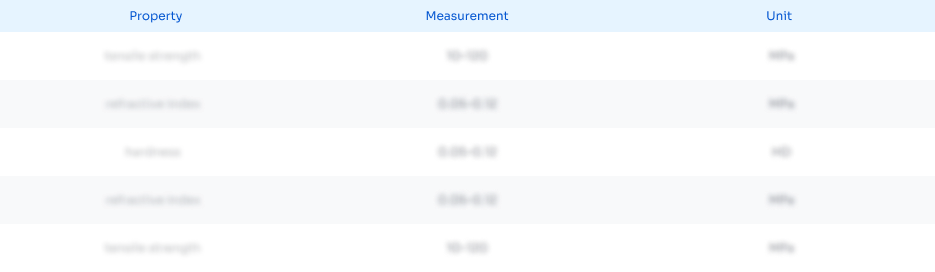
Abstract
Description
Claims
Application Information
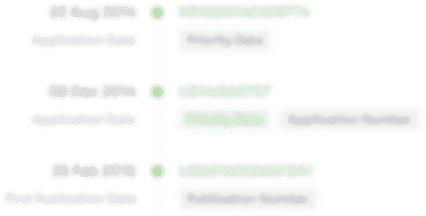
- R&D
- Intellectual Property
- Life Sciences
- Materials
- Tech Scout
- Unparalleled Data Quality
- Higher Quality Content
- 60% Fewer Hallucinations
Browse by: Latest US Patents, China's latest patents, Technical Efficacy Thesaurus, Application Domain, Technology Topic, Popular Technical Reports.
© 2025 PatSnap. All rights reserved.Legal|Privacy policy|Modern Slavery Act Transparency Statement|Sitemap|About US| Contact US: help@patsnap.com