Aircraft full-automatic pneumatic optimization method based on reinforcement learning and transfer learning
A technology of reinforcement learning and transfer learning, applied in the field of aircraft engineering, can solve the problems of incompatibility between global optimization ability and convergence speed, and achieve the effect of quickly obtaining high-precision aircraft design parameters, increasing convergence speed, and improving iteration efficiency
- Summary
- Abstract
- Description
- Claims
- Application Information
AI Technical Summary
Problems solved by technology
Method used
Image
Examples
Embodiment Construction
[0023] The following examples are used to further illustrate the present invention. The software, file format and platform described here are used to provide a further understanding of the present invention, but do not therefore limit the protection scope of the present invention to the scope described in the examples.
[0024] Firstly, the missile is selected as the object to optimize the aerodynamic shape of its wings. When optimizing the target, the lift-to-drag ratio is increased under the premise of keeping the aerodynamic center basically unchanged. The chord length, wing root chord length and the sweep angle of the leading and trailing edges of the airfoil are optimized as design parameters.
[0025] Then the reinforcement learning environment based on the semi-empirical estimation software DATCOM and the high-precision fluid simulation software Fluent is respectively established. For DATCOM, use Python language to write calculation files, which include flight condition...
PUM
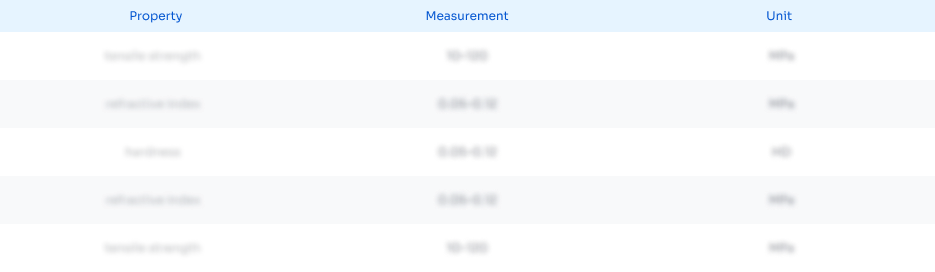
Abstract
Description
Claims
Application Information
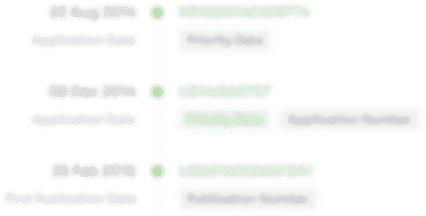
- R&D
- Intellectual Property
- Life Sciences
- Materials
- Tech Scout
- Unparalleled Data Quality
- Higher Quality Content
- 60% Fewer Hallucinations
Browse by: Latest US Patents, China's latest patents, Technical Efficacy Thesaurus, Application Domain, Technology Topic, Popular Technical Reports.
© 2025 PatSnap. All rights reserved.Legal|Privacy policy|Modern Slavery Act Transparency Statement|Sitemap|About US| Contact US: help@patsnap.com