A particle image velocimetry method based on convolutional neural network
A technology of convolutional neural network and particle image speed measurement, which is applied in the field of particle image speed measurement based on convolutional neural network, can solve the problems of increasing the calculation amount of correlation analysis method, and achieve the effect of reducing calculation time, high precision and improving calculation efficiency
- Summary
- Abstract
- Description
- Claims
- Application Information
AI Technical Summary
Problems solved by technology
Method used
Image
Examples
Embodiment Construction
[0035] The present invention will be described in further detail below in conjunction with the accompanying drawings and specific embodiments.
[0036] The invention provides a particle image velocity measurement method based on a convolutional neural network, which is characterized in that the method uses a supervised deep learning convolutional neural network (Convolutional Neural Network, CNN) to extract and analyze the velocity field from a two-dimensional fluid particle image . figure 1 For the realization flowchart of the inventive method, it comprises the following steps:
[0037] Step 1: Generate PIV dataset;
[0038] The PIV training data set refers to a large number of artificially generated particle images and corresponding velocity field labels, which are used for the training of convolutional neural networks. Each data item used for training in the data set contains two consecutive frames of particle images f 1 ,f 2 and a velocity vector field ω.
[0039] In ...
PUM
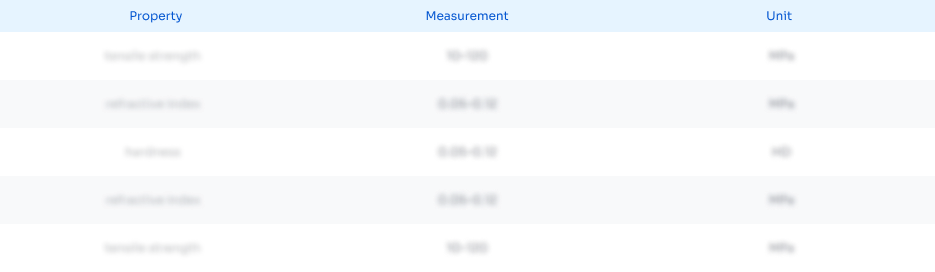
Abstract
Description
Claims
Application Information
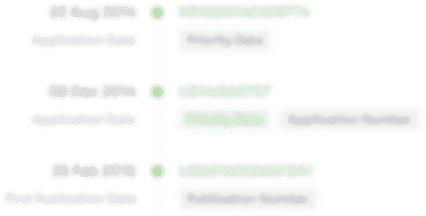
- R&D
- Intellectual Property
- Life Sciences
- Materials
- Tech Scout
- Unparalleled Data Quality
- Higher Quality Content
- 60% Fewer Hallucinations
Browse by: Latest US Patents, China's latest patents, Technical Efficacy Thesaurus, Application Domain, Technology Topic, Popular Technical Reports.
© 2025 PatSnap. All rights reserved.Legal|Privacy policy|Modern Slavery Act Transparency Statement|Sitemap|About US| Contact US: help@patsnap.com