A road image semantic segmentation method based on a hybrid automatic encoder
A semantic segmentation and hybrid automatic technology, applied in the field of computer vision, can solve problems such as training difficulties, real-time impact, and complex network structure of road semantic segmentation models, and achieve the effects of shortening the training cycle, high segmentation accuracy, and easy convergence
- Summary
- Abstract
- Description
- Claims
- Application Information
AI Technical Summary
Problems solved by technology
Method used
Image
Examples
Embodiment Construction
[0022] Such as figure 1 As shown, the present invention is a road image semantic segmentation method based on a hybrid autoencoder, comprising the following steps:
[0023] (10) sample set collection: the collected sample set images are divided into training sample set and test sample set images;
[0024] The (10) sample collection step comprises:
[0025] (11) Sample collection: The Cambridge-driving Labeled VideoDatabase (CamVid) dataset publicly available on the Internet was selected. The CamVid dataset is a road and driving scene understanding dataset. The collection of the dataset comes from a camera with a resolution of 960×720 pixels installed on the dashboard of a car;
[0026] (12) Classification of sample sets: the training sample set is used to train the model, the test sample set is used to test the model, and the approximate number ratio is 4:1;
[0027] (20) Sample image preprocessing: perform size transformation and contrast normalization processing on the sa...
PUM
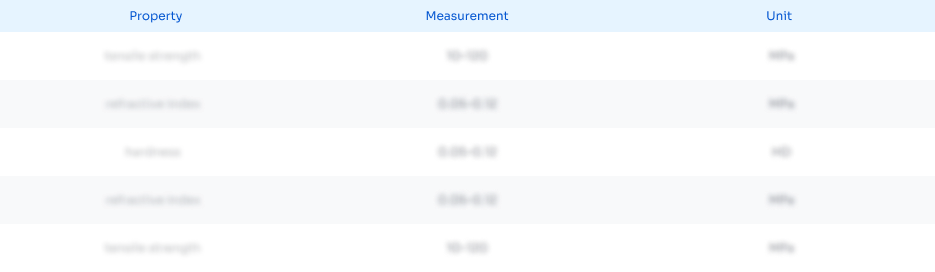
Abstract
Description
Claims
Application Information
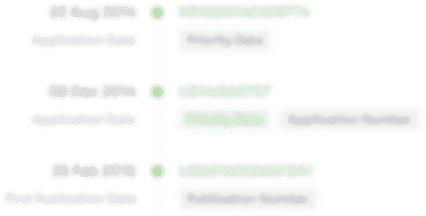
- R&D
- Intellectual Property
- Life Sciences
- Materials
- Tech Scout
- Unparalleled Data Quality
- Higher Quality Content
- 60% Fewer Hallucinations
Browse by: Latest US Patents, China's latest patents, Technical Efficacy Thesaurus, Application Domain, Technology Topic, Popular Technical Reports.
© 2025 PatSnap. All rights reserved.Legal|Privacy policy|Modern Slavery Act Transparency Statement|Sitemap|About US| Contact US: help@patsnap.com