An abdominal muscle labeling method and device based on deep learning
A deep learning and muscle technology, applied in the field of medical image processing and artificial intelligence, to achieve the effect of strong practicability, accurate data, and guaranteed accuracy
- Summary
- Abstract
- Description
- Claims
- Application Information
AI Technical Summary
Problems solved by technology
Method used
Image
Examples
Embodiment 1
[0057] See figure 1 , figure 1 It is a flowchart of a method for labeling abdominal muscles based on deep learning in the present invention, and the method for labeling abdominal muscles based on deep learning includes the following steps:
[0058] Step 1: Collect Data
[0059] The purpose of this step is to collect abdominal CT image data and guide doctors to collect abdominal CT image data including the third lumbar vertebra. Each CT image contains 5-6 scans of the third lumbar spine.
[0060] In this step, CT data with poor quality (the image of the third lumbar vertebra is too blurred) will be eliminated.
[0061] Step 2: Label the data
[0062] The purpose of this step is to label the abdominal CT image data collected in the first step, and then train the segmentation model. This step includes two sub-steps: marking the position of the third lumbar vertebra and marking muscle groups.
[0063] Mark the position of the third lumbar vertebra, that is, mark the starting...
Embodiment 2
[0079] The abdominal muscle labeling method based on deep learning provided in this embodiment specifically includes the following steps:
[0080] Step 1: Collect Data
[0081] The purpose of this step is to collect abdominal CT image data and guide doctors to collect abdominal CT image data including the third lumbar vertebra. Each CT image contains 5-6 scans of the third lumbar spine.
[0082] In this step, CT data with poor quality (the image of the third lumbar vertebra is too blurred) will be eliminated.
[0083] Step 2: Label the data
[0084] The purpose of this step is to label the abdominal CT image data collected in the first step, and then train the segmentation model. This step includes two sub-steps: marking the position of the third lumbar vertebra and marking muscle groups.
[0085] Mark the position of the third lumbar vertebra, that is, mark the starting CT page number of the third lumbar vertebra. This information will be used in validation testing of th...
Embodiment 3
[0109] See Figure 5 , Figure 5 It is a structural block diagram of an abdominal muscle labeling device based on deep learning in the present invention, and the abdominal muscle labeling device based on deep learning includes:
[0110] The image acquisition module 1 is configured to acquire abdominal CT image data including the third lumbar vertebra.
[0111] An annotation module 2 is configured to annotate the abdominal CT image data acquired by the image acquisition module 1 . The marking module 2 further includes two sub-modules, specifically the third lumbar position marking sub-module 21 and the muscle group marking sub-module 22, the third lumbar position marking sub-module 21 is used to mark the third lumbar position, that is, marking the third lumbar position. The starting CT page number of the three lumbar vertebrae. This information will be used in validation testing of the segmentation model. The muscle group labeling sub-module 22 is used for labeling muscle g...
PUM
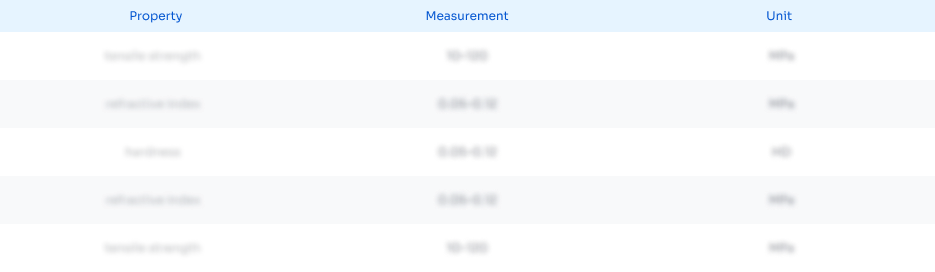
Abstract
Description
Claims
Application Information
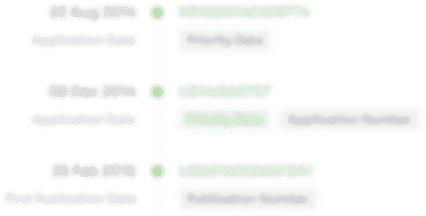
- R&D Engineer
- R&D Manager
- IP Professional
- Industry Leading Data Capabilities
- Powerful AI technology
- Patent DNA Extraction
Browse by: Latest US Patents, China's latest patents, Technical Efficacy Thesaurus, Application Domain, Technology Topic, Popular Technical Reports.
© 2024 PatSnap. All rights reserved.Legal|Privacy policy|Modern Slavery Act Transparency Statement|Sitemap|About US| Contact US: help@patsnap.com