A fine-grained classification method based on meta-learning
A classification method and fine-grained technology, applied in the field of calculation and calculation, can solve the problem of not having large data sets, and achieve the effect of reducing the number of parameters and the amount of calculation, strong robustness, and improving network performance
- Summary
- Abstract
- Description
- Claims
- Application Information
AI Technical Summary
Problems solved by technology
Method used
Image
Examples
Embodiment Construction
[0024] The present invention provides a kind of fine-grained classification method based on meta-learning, comprising the following steps:
[0025] Establish an external dataset: establish an external dataset based on the public fine-grained classification database of the research institution or self-collected data. For example, the fine-grained classification database can choose Caltech-UCSD Brids 200 (CUB-200) dataset or DogNet. Each image should include an identification label indicating which category the image belongs to. As many individuals as possible should be collected, each individual contains as many samples as possible, while reducing the number of mislabeled samples in the dataset. The increase in the number of samples and the number of categories will improve the training accuracy; the sample categories of the test set are smaller than the training set, and the sample categories of the test set and the training set are completely disjoint. A large data set can b...
PUM
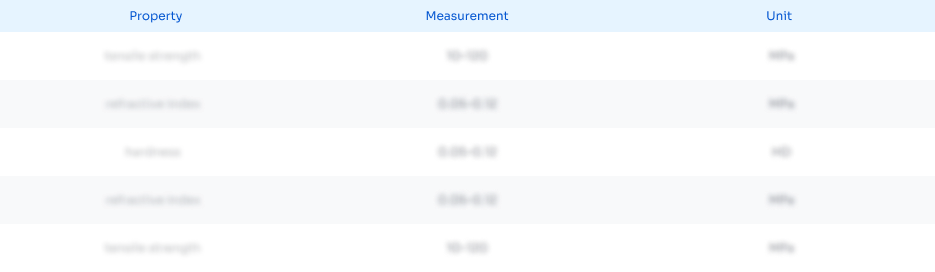
Abstract
Description
Claims
Application Information
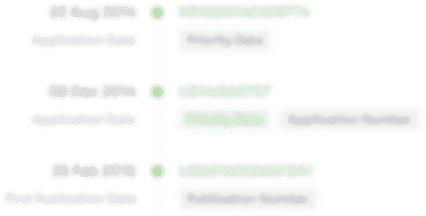
- R&D Engineer
- R&D Manager
- IP Professional
- Industry Leading Data Capabilities
- Powerful AI technology
- Patent DNA Extraction
Browse by: Latest US Patents, China's latest patents, Technical Efficacy Thesaurus, Application Domain, Technology Topic, Popular Technical Reports.
© 2024 PatSnap. All rights reserved.Legal|Privacy policy|Modern Slavery Act Transparency Statement|Sitemap|About US| Contact US: help@patsnap.com