A d2d resource allocation method based on multi-agent deep reinforcement learning
A technology of reinforcement learning and resource allocation, applied in the field of D2D resource allocation based on multi-agent deep reinforcement learning, can solve problems such as same-layer interference, D2D communication interference management, and cross-layer interference of cellular communication links
- Summary
- Abstract
- Description
- Claims
- Application Information
AI Technical Summary
Problems solved by technology
Method used
Image
Examples
Embodiment Construction
[0071] In order to make the technical principles of the present invention more clearly understood, the embodiments of the present invention will be described in detail below in conjunction with the accompanying drawings.
[0072] A D2D resource allocation method based on multi-agent deep reinforcement learning (MADRL, Multi-Agent Deep Reinforcement Learning based Device-to-Device Resource Allocation Method) is applied to the heterogeneous network where the cellular network and D2D communication coexist; first establish the D2D respectively The expression of signal-to-interference-noise ratio and communication rate per unit bandwidth of receiving users and cellular users, with the optimization goal of maximizing system capacity, taking the SINR of cellular users greater than the minimum SINR threshold, D2D link spectrum allocation constraints and the emission of D2D transmitting users The power is less than the maximum transmit power threshold as the optimization condition, and ...
PUM
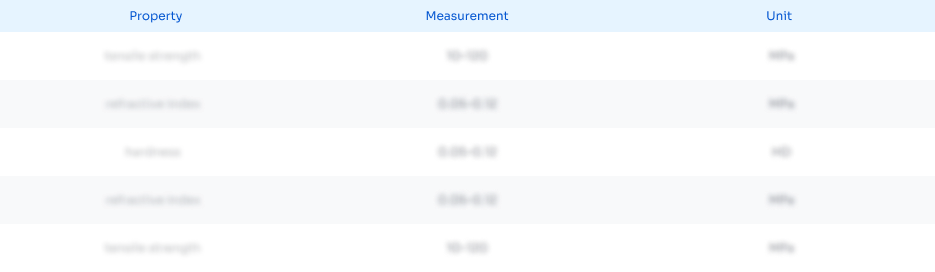
Abstract
Description
Claims
Application Information
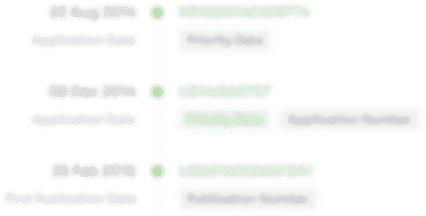
- R&D Engineer
- R&D Manager
- IP Professional
- Industry Leading Data Capabilities
- Powerful AI technology
- Patent DNA Extraction
Browse by: Latest US Patents, China's latest patents, Technical Efficacy Thesaurus, Application Domain, Technology Topic, Popular Technical Reports.
© 2024 PatSnap. All rights reserved.Legal|Privacy policy|Modern Slavery Act Transparency Statement|Sitemap|About US| Contact US: help@patsnap.com