A Method for Predicting the Running Time of Bus Sections Based on Improved Deep Forest
A technology of running time and prediction method, which is applied in the direction of prediction, traffic flow detection, traffic control system of road vehicles, etc. It can solve the problems of dependence on the parameter adjustment process, many hyperparameters, and complex models, so as to reduce the parameter adjustment process and increase the The effect of large dimensionality and strong representation learning ability
- Summary
- Abstract
- Description
- Claims
- Application Information
AI Technical Summary
Problems solved by technology
Method used
Image
Examples
Embodiment
[0039] The invention provides a method for predicting the running time of a bus section based on an improved deep forest, comprising the steps of:
[0040] Step S1: Collect bus line information, GPS information, and road section information, and normalize the collected information data, and process the collected data into data between [0,1] using the following formula:
[0041]
[0042] Among them, w is the normalized data; x is the original data; x min is the minimum value in the original data; x max is the maximum value in the original data;
[0043] Step S2: Input the processed data into the improved deep forest for training, the improved deep forest consists of two parts: convolutional multi-grained scanning and limited cascade forest;
[0044] Such as figure 1 , 2 As shown, convolutional multi-grained scanning is equivalent to feature extraction for selection among raw features. When sliding window scanning to select sample features, a column of vectors (similar t...
PUM
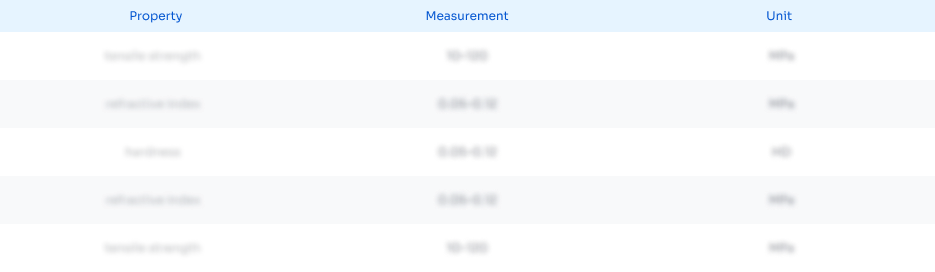
Abstract
Description
Claims
Application Information
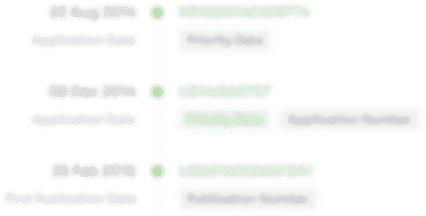
- R&D Engineer
- R&D Manager
- IP Professional
- Industry Leading Data Capabilities
- Powerful AI technology
- Patent DNA Extraction
Browse by: Latest US Patents, China's latest patents, Technical Efficacy Thesaurus, Application Domain, Technology Topic, Popular Technical Reports.
© 2024 PatSnap. All rights reserved.Legal|Privacy policy|Modern Slavery Act Transparency Statement|Sitemap|About US| Contact US: help@patsnap.com