Pedestrian re-identification method fusing random batch masks and multi-scale representation learning
A pedestrian re-identification, multi-scale technology, applied in neural learning methods, character and pattern recognition, computer components, etc., can solve the problems that the subsequent recognition accuracy cannot be guaranteed, and important detailed features cannot be effectively extracted, etc., to achieve Guarantee recognition accuracy, save network expenses, and improve accuracy
- Summary
- Abstract
- Description
- Claims
- Application Information
AI Technical Summary
Problems solved by technology
Method used
Image
Examples
Embodiment
[0053] Such as figure 1 As shown, a person re-identification method that combines random batch masks and multi-scale representation learning includes the following steps:
[0054] S1. Obtain a benchmark data set, and perform data expansion on the benchmark data set;
[0055] S2. Divide the benchmark data set after data expansion into a training set and a test set;
[0056] S3. Based on the ResNet50 convolutional neural network, construct a pedestrian re-identification training network that includes sequentially connected attention learning modules, feature extraction modules, and recognition output modules. The feature extraction module includes feature processing branches, multi-scale representation learning branches and random A batch mask branch, the feature processing branch includes global average pooling and batch normalization processing;
[0057] S4. Input the training set into the pedestrian re-identification training network, adjust the network hyperparameters acco...
PUM
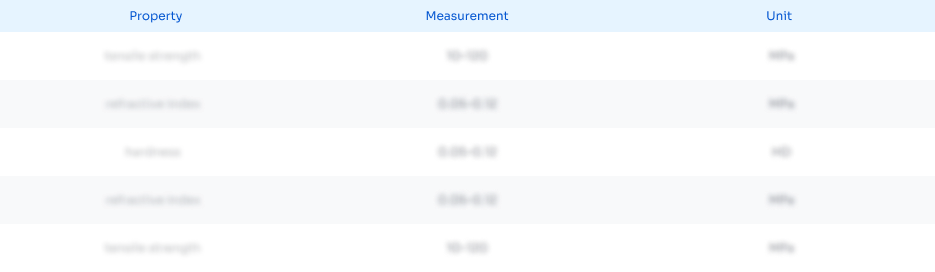
Abstract
Description
Claims
Application Information
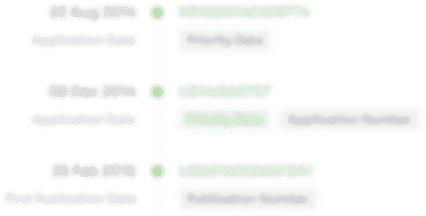
- R&D Engineer
- R&D Manager
- IP Professional
- Industry Leading Data Capabilities
- Powerful AI technology
- Patent DNA Extraction
Browse by: Latest US Patents, China's latest patents, Technical Efficacy Thesaurus, Application Domain, Technology Topic, Popular Technical Reports.
© 2024 PatSnap. All rights reserved.Legal|Privacy policy|Modern Slavery Act Transparency Statement|Sitemap|About US| Contact US: help@patsnap.com