Motor imagery electroencephalogram signal classification method based on deep learning
An EEG signal and motor imagery technology, applied in the field of pattern recognition and brain-computer interface, can solve the problems of slow speed, high requirements for EEG signal preprocessing, and insufficient classification accuracy, so as to reduce redundancy and improve classification Efficiency, fast learning effect
- Summary
- Abstract
- Description
- Claims
- Application Information
AI Technical Summary
Problems solved by technology
Method used
Image
Examples
Embodiment
[0050] see figure 1 , the classification method of the motor imagery EEG signal based on deep learning of the present embodiment, comprises the following steps:
[0051]Step 1: Acquisition of experimental data, that is, collecting the original EEG signal data set, the subject wears an electrode cap during collection, and the original EEG signal is performed through the 64-lead EEG amplifier of the 10-10 method calibrated by the International Electroencephalography Association Collect and select all electrode positions. Each subject will conduct 14 experiments. When collecting, the subject sits on a chair, and there is a screen in front of the subject, and there is a target on the screen: the first two experiments It is a basic experiment lasting 1 minute. The basic experiment includes opening and closing the eyes, that is, in the first two experiments, the eyes were opened for 1 minute and the eyes were closed for 1 minute; then there were three cycles of experiments, and each...
PUM
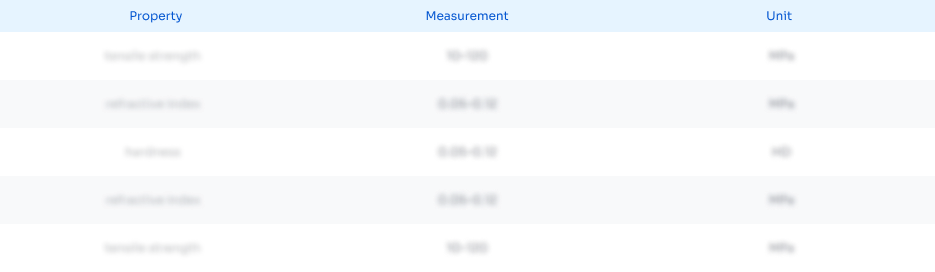
Abstract
Description
Claims
Application Information
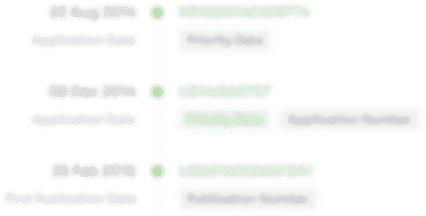
- R&D
- Intellectual Property
- Life Sciences
- Materials
- Tech Scout
- Unparalleled Data Quality
- Higher Quality Content
- 60% Fewer Hallucinations
Browse by: Latest US Patents, China's latest patents, Technical Efficacy Thesaurus, Application Domain, Technology Topic, Popular Technical Reports.
© 2025 PatSnap. All rights reserved.Legal|Privacy policy|Modern Slavery Act Transparency Statement|Sitemap|About US| Contact US: help@patsnap.com