Super-resolution reconstruction method based on a fused multi-level feature map
A super-resolution reconstruction and feature map technology, applied in the field of computer vision, can solve problems such as inconsistency in subjective evaluation and achieve good accuracy
- Summary
- Abstract
- Description
- Claims
- Application Information
AI Technical Summary
Problems solved by technology
Method used
Image
Examples
Embodiment Construction
[0029] Embodiments of the invention are described in detail below, examples of which are illustrated in the accompanying drawings. The embodiments described below by referring to the figures are exemplary only for explaining the present invention and should not be construed as limiting the present invention.
[0030] Such as figure 1 As shown, a super-resolution reconstruction method based on convolutional neural network, the network model is divided into two parts: feature extraction network and reconstruction network. The feature extraction network can be divided into a feature extraction part and a feature fusion part. The feature extraction part uses t identical convolutional layers, and the feature fusion part consists of a 1×1CNN and a 3×3CNN. The reconstruction network consists of an upsampling operator and a convolutional layer.
[0031] Specific steps are as follows:
[0032] Step 1: Use sequentially connected convolutional layers to extract features from low-resol...
PUM
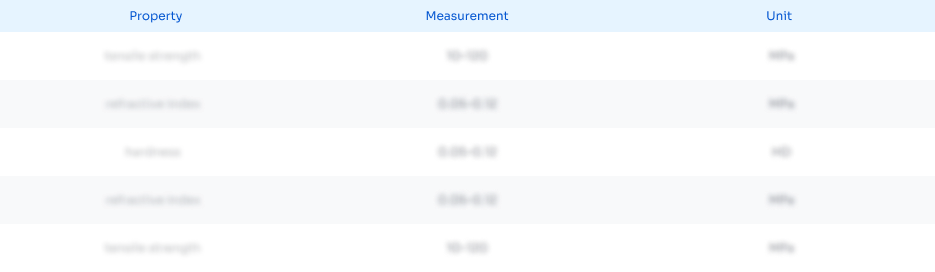
Abstract
Description
Claims
Application Information
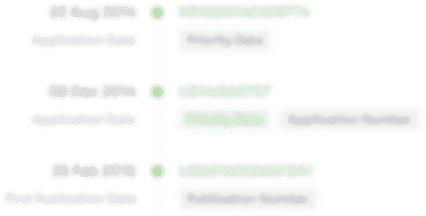
- R&D Engineer
- R&D Manager
- IP Professional
- Industry Leading Data Capabilities
- Powerful AI technology
- Patent DNA Extraction
Browse by: Latest US Patents, China's latest patents, Technical Efficacy Thesaurus, Application Domain, Technology Topic, Popular Technical Reports.
© 2024 PatSnap. All rights reserved.Legal|Privacy policy|Modern Slavery Act Transparency Statement|Sitemap|About US| Contact US: help@patsnap.com