Pipeline fault diagnosis method based on convolutional neural network
A convolutional neural network and fault diagnosis technology, applied in the field of pipeline fault diagnosis based on convolutional neural network, can solve the problems of high misjudgment and low efficiency of pipeline fault detection, and achieve the effect of improving the ability of identification and diagnosis
- Summary
- Abstract
- Description
- Claims
- Application Information
AI Technical Summary
Problems solved by technology
Method used
Image
Examples
Embodiment Construction
[0032] The present invention will be described in detail in conjunction with accompanying drawing now. This figure is a simplified schematic diagram only illustrating the basic structure of the present invention in a schematic manner, so it only shows the components relevant to the present invention.
[0033] A pipeline fault diagnosis method based on convolutional neural network of the present invention, such as figure 1 As shown, it is a classification flowchart of the method of the present invention, and the forward and backward training steps in the classification flow are as follows:
[0034] S1: Signal collection and processing: apply excitation to the pipeline to be tested, and collect excitation response signals at the test points of the pipeline;
[0035] S2: After denoising the collected pipeline fault signal, normalize it and extract the fault characteristic signal to obtain the pipeline fault characteristic signal, and divide the processed signal into training sam...
PUM
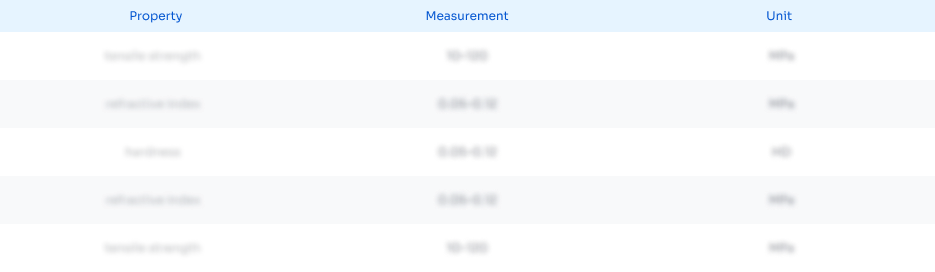
Abstract
Description
Claims
Application Information
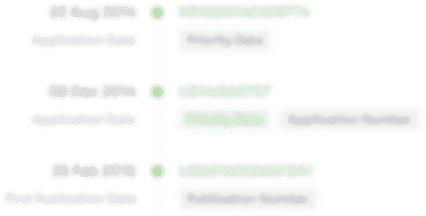
- R&D Engineer
- R&D Manager
- IP Professional
- Industry Leading Data Capabilities
- Powerful AI technology
- Patent DNA Extraction
Browse by: Latest US Patents, China's latest patents, Technical Efficacy Thesaurus, Application Domain, Technology Topic, Popular Technical Reports.
© 2024 PatSnap. All rights reserved.Legal|Privacy policy|Modern Slavery Act Transparency Statement|Sitemap|About US| Contact US: help@patsnap.com