Smart city traffic signal control recommendation method, system and device
A signal control and traffic signal technology, applied in the field of smart city traffic signal control recommendation, can solve the problems of low efficiency and low reliability
- Summary
- Abstract
- Description
- Claims
- Application Information
AI Technical Summary
Problems solved by technology
Method used
Image
Examples
Embodiment approach 1
[0046] A kind of implementation mode 1, refer to image 3 , LSTM can solve the problem of long-term and short-term dependence. LSTM includes three gate designs, namely: input gate, forget gate and output gate. The input gate processes the input information at the current moment and is responsible for passing instant information to the memory. The cell; the forget gate processes the long-term state and is responsible for continuing to store long-term information; the output gate processes the current cell state and is responsible for controlling the long-term state as the output of the current LSTM. The data of each period is processed in turn, and the model parameters are updated by using the backpropagation of the error, and then the information of the specified period in the future is estimated. The formula of the gate is as follows, where σ is the activation function, W is the change matrix, and X t is the input parameter, b is the bias function:
[0047] g(X)=σ(WX t +b)...
Embodiment approach 2
[0052] A kind of implementation mode 2, refer to Figure 4 , RNN algorithm process: x t Indicates the information input at time t, s t Represents the hidden state of the model at sequence index t. the s t by x t and s t-1 jointly decide; o t Represents the output of the model at sequence index t. o t Only determined by the model's current hidden state st. The two matrices U and W are respectively x in the RNN network t and s t Linear relationship parameter, V is s t The parameters of the output layer. It is shared across the entire RNN network. The RNN loop processes the data of each cycle, backpropagates the error to update the parameter value, and estimates the information of the future cycle. The algorithm formula is as follows, where W represents the weight from the hidden layer to the hidden layer, and U represents the weight from the input layer to the hidden layer. The weight of the hidden layer, V represents the weight from the hidden layer to the output l...
Embodiment approach 3
[0061] A kind of embodiment 3, deep learning algorithm model selection process, deep learning algorithm N, refer to embodiment 1, embodiment 2, training obtains N deep learning algorithm models, compares model performance, selects and satisfies according to preset model performance requirements A deep learning algorithm model for model performance requirements. Model performance includes but is not limited to: the target value at the end of model training, the time required for model training, the output performance of the model applied to a new data set, etc.
PUM
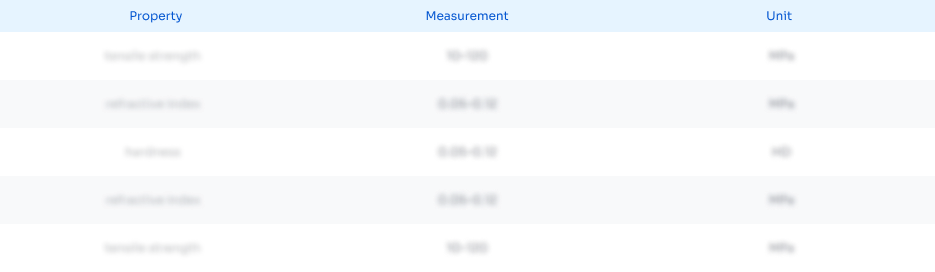
Abstract
Description
Claims
Application Information
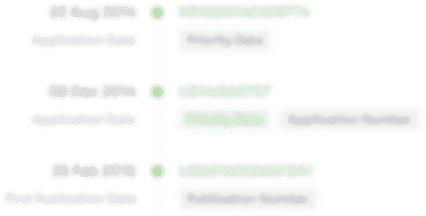
- Generate Ideas
- Intellectual Property
- Life Sciences
- Materials
- Tech Scout
- Unparalleled Data Quality
- Higher Quality Content
- 60% Fewer Hallucinations
Browse by: Latest US Patents, China's latest patents, Technical Efficacy Thesaurus, Application Domain, Technology Topic, Popular Technical Reports.
© 2025 PatSnap. All rights reserved.Legal|Privacy policy|Modern Slavery Act Transparency Statement|Sitemap|About US| Contact US: help@patsnap.com