An order production progress real-time prediction method based on double-layer transfer learning
A technology for transfer learning and production progress, applied in neural learning methods, prediction, biological neural network models, etc., can solve problems such as low prediction efficiency, poor generalization performance, and low prediction accuracy, to improve prediction accuracy and meet online analysis. The effect of forecasting and improving budget efficiency
- Summary
- Abstract
- Description
- Claims
- Application Information
AI Technical Summary
Problems solved by technology
Method used
Image
Examples
Embodiment Construction
[0045] The drawings constituting a part of the present invention are used to provide a further understanding of the present invention, and the schematic embodiments and descriptions of the present invention are used to explain the present invention, and do not constitute an improper limitation of the present invention.
[0046] This embodiment provides a method for real-time forecasting of order production progress based on two-layer transfer learning. The specific implementation method is as follows: figure 1 As shown, it includes the following steps:
[0047] Step (1): Using IoT sensing equipment deployed in the workshop to collect massive production data (manufacturing data) of multiple continuous production processes in time series, the massive production data includes order task data OT, task data CT completed at the current moment, and real-time production data. State data RC and forecast time ΔT; and form a data set characterized by data OT, CT, RC and ΔT, and divide th...
PUM
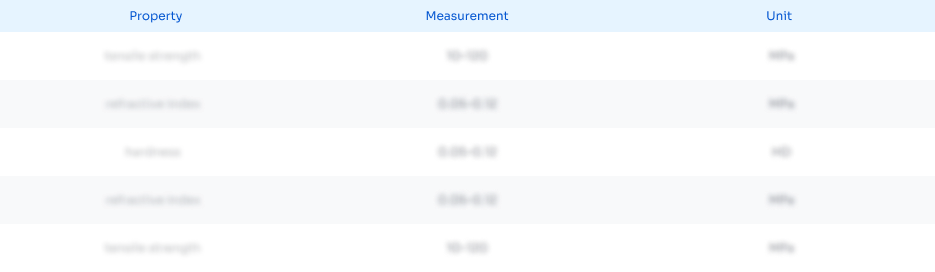
Abstract
Description
Claims
Application Information
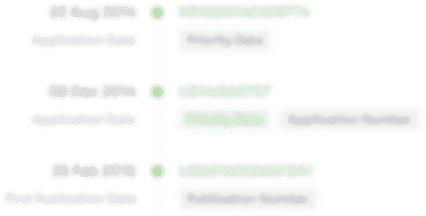
- R&D Engineer
- R&D Manager
- IP Professional
- Industry Leading Data Capabilities
- Powerful AI technology
- Patent DNA Extraction
Browse by: Latest US Patents, China's latest patents, Technical Efficacy Thesaurus, Application Domain, Technology Topic.
© 2024 PatSnap. All rights reserved.Legal|Privacy policy|Modern Slavery Act Transparency Statement|Sitemap