Multi-decoder full convolutional neural network and a corresponding mesoscopic structure identification method thereof
A convolutional neural network and mesoscopic structure technology, applied in the field of multi-decoder full convolutional neural network, can solve problems such as enhancing the robustness of algorithms, fuzzy edges of feature maps, and loss of content information, so as to improve accuracy and reduce Error resolution, wide range of effects
- Summary
- Abstract
- Description
- Claims
- Application Information
AI Technical Summary
Problems solved by technology
Method used
Image
Examples
Embodiment Construction
[0038] The present invention is described in further detail now in conjunction with accompanying drawing.
[0039] Such as figure 1 As shown, the present invention proposes a multi-decoder fully convolutional neural network and a method for recognizing the microstructure of ceramic matrix composite material preforms based on semantic segmentation of the network, including the following steps:
[0040] 1. Construct an XCT image dataset for training ceramic matrix composites.
[0041] A series of XCT slices and truth maps of complex ceramic matrix composite preforms are collected to construct a semantic segmentation dataset Q for training a multi-decoder fully convolutional deep network.
[0042] Second, the preprocessing of data enhancement for the semantic segmentation dataset makes the image dataset more comprehensive.
[0043] Data enhancement preprocessing methods specifically include cropping, scaling, rotation, brightness change, and contrast enhancement.
[0044] 3. S...
PUM
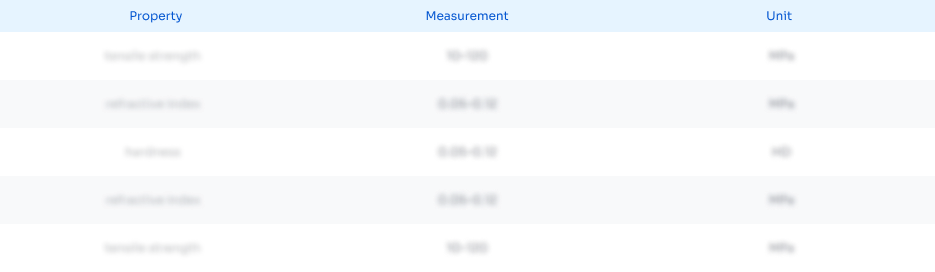
Abstract
Description
Claims
Application Information
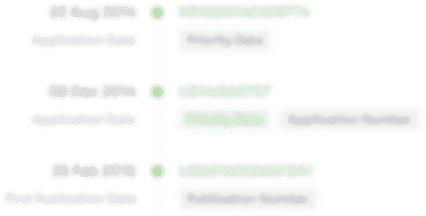
- R&D Engineer
- R&D Manager
- IP Professional
- Industry Leading Data Capabilities
- Powerful AI technology
- Patent DNA Extraction
Browse by: Latest US Patents, China's latest patents, Technical Efficacy Thesaurus, Application Domain, Technology Topic.
© 2024 PatSnap. All rights reserved.Legal|Privacy policy|Modern Slavery Act Transparency Statement|Sitemap