A High-speed Ramp Travel Time Prediction Method Based on Multi-model Fusion
A technology of travel time and prediction method, applied in the field of machine learning, which can solve problems such as weak generalization ability, poor stability of prediction effect, and complex parameter setting of a single prediction model
- Summary
- Abstract
- Description
- Claims
- Application Information
AI Technical Summary
Problems solved by technology
Method used
Image
Examples
Embodiment
[0037] like figure 1 As shown, this embodiment provides a high-speed ramp travel time prediction method based on multi-model fusion, which specifically includes:
[0038] like figure 2 As shown, use the following steps to obtain multiple pre-trained weak learners:
[0039] B1. Using the self-service sampling method to obtain multiple sample sampling sets for the training sample set;
[0040] B2. Perform data processing for each sample sampling set to obtain training samples suitable for each learning model;
[0041] B3. Using the training samples to train a corresponding learning model and obtain multiple pre-trained weak learners.
[0042] For example, this embodiment selects XGBoost (hereinafter referred to as Xgb), LightGBM, SVM, linear regression (Linear regression) and KNN as multiple pre-trained weak learner models to include.
[0043] S1. Obtain historical driving time data, and directly map the historical driving time data to obtain the first prediction result SWL...
PUM
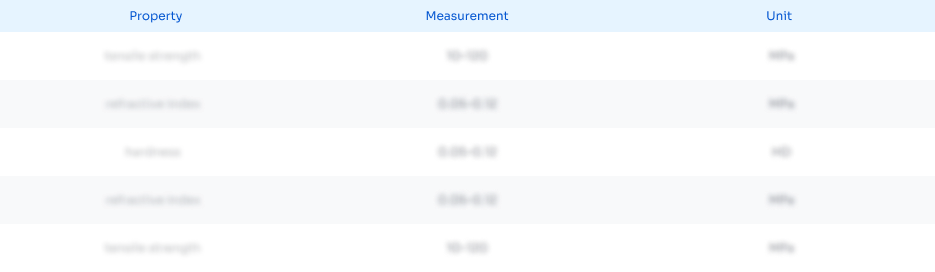
Abstract
Description
Claims
Application Information
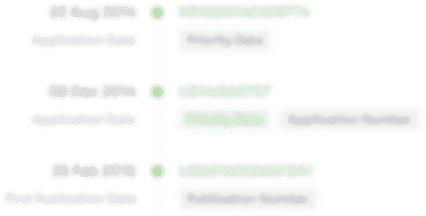
- R&D Engineer
- R&D Manager
- IP Professional
- Industry Leading Data Capabilities
- Powerful AI technology
- Patent DNA Extraction
Browse by: Latest US Patents, China's latest patents, Technical Efficacy Thesaurus, Application Domain, Technology Topic, Popular Technical Reports.
© 2024 PatSnap. All rights reserved.Legal|Privacy policy|Modern Slavery Act Transparency Statement|Sitemap|About US| Contact US: help@patsnap.com