Residual error network deep learning method for mechanical arm grabbing pose estimation
A robotic arm and residual technology, applied in neural learning methods, biological neural network models, calculations, etc., can solve problems such as reducing the recognition accuracy of neural networks, and achieve the effect of improving accuracy
- Summary
- Abstract
- Description
- Claims
- Application Information
AI Technical Summary
Problems solved by technology
Method used
Image
Examples
Embodiment Construction
[0077] In order to better explain the present invention and facilitate understanding, the present invention will be described in detail below through specific embodiments in conjunction with the accompanying drawings.
[0078] Autonomous grasping of manipulators is an important issue in the field of robotics research. For the optimal grasping pose problem, this application endows the robotic arm with vision and combines deep learning algorithms to realize the intelligence of the robotic arm's grasping.
[0079] In this application, the idea of residual network is adopted to improve the grasping and generating convolutional neural network (GG-CNN). First, the convolutional residual module (such as Figure 9 As shown), the residual network is constructed by multi-layer stacking of the residual module, and the depth of the convolutional neural network is deepened, and this is used as the main part of improving GG-CNN. In this application, the GG-CNN is improved through the dee...
PUM
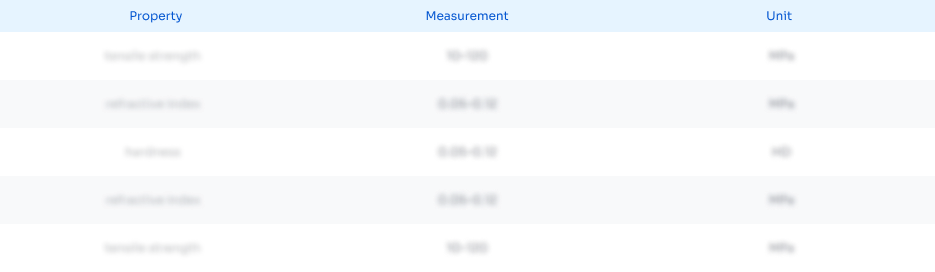
Abstract
Description
Claims
Application Information
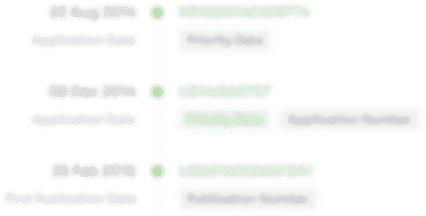
- R&D Engineer
- R&D Manager
- IP Professional
- Industry Leading Data Capabilities
- Powerful AI technology
- Patent DNA Extraction
Browse by: Latest US Patents, China's latest patents, Technical Efficacy Thesaurus, Application Domain, Technology Topic, Popular Technical Reports.
© 2024 PatSnap. All rights reserved.Legal|Privacy policy|Modern Slavery Act Transparency Statement|Sitemap|About US| Contact US: help@patsnap.com