Representative image selection method based on multi-example active learning
An active learning, representational technique used in instrumentation, character and pattern recognition, computer components, etc.
- Summary
- Abstract
- Description
- Claims
- Application Information
AI Technical Summary
Problems solved by technology
Method used
Image
Examples
Embodiment Construction
[0044] The present invention will be described in detail below in conjunction with the embodiments and accompanying drawings, but the present invention is not limited thereto. The active learning framework refers to first using the initial sample to train the initial classifier, and using the initial classifier to predict all unlabeled samples in the original sample set; The samples with a large contribution to the classification accuracy of the classifier are handed over to the labeling experts for labeling; then the labeled samples are used together with the initial samples to train the classifier. Iterate in this way until the accuracy of the classifier does not change. At this time, although the classifier only uses a small number of labeled samples, it can achieve better classification accuracy.
[0045] The COCO data set is a target detection and classification data set provided by Microsoft. The images in the data set are mainly intercepted from complex daily scenes. Th...
PUM
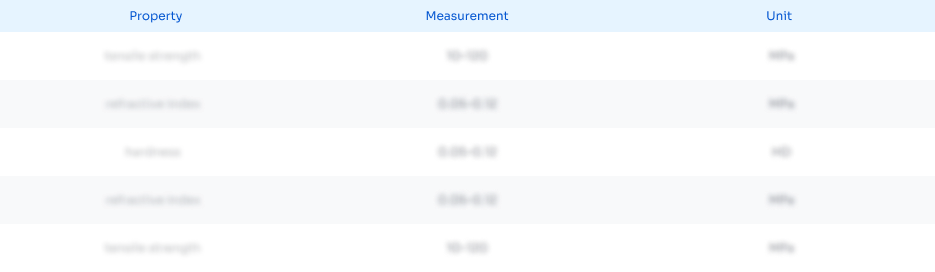
Abstract
Description
Claims
Application Information
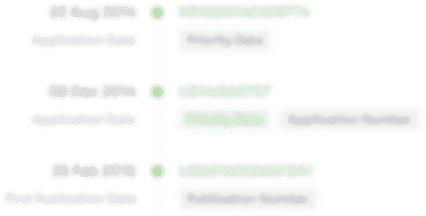
- R&D Engineer
- R&D Manager
- IP Professional
- Industry Leading Data Capabilities
- Powerful AI technology
- Patent DNA Extraction
Browse by: Latest US Patents, China's latest patents, Technical Efficacy Thesaurus, Application Domain, Technology Topic, Popular Technical Reports.
© 2024 PatSnap. All rights reserved.Legal|Privacy policy|Modern Slavery Act Transparency Statement|Sitemap|About US| Contact US: help@patsnap.com